Reproducibility
Reproducibility is the closeness of the agreement between the results of measurements of the same measurand carried out with the same methodology described in the corresponding scientific evidence (e.g. a publication in a peer-reviewed journal).[1] Reproducibility can also be applied under changed conditions of measurement for the same measurand—to check that the results are not an artefact of the measurement procedures.[2][3]
A related concept is replication, which is the ability to independently achieve non-identical conclusions that are at least similar, when differences in sampling, research procedures and data analysis methods may exist.[4] Reproducibility and replicability together are among the main tools of the scientific method.[5] However, the concrete expressions of the ideal of the scientific method vary considerably across research disciplines and fields of study. The study of reproducibility is an important topic in metascience.[6]
About
The values obtained from distinct experimental trials are said to be "commensurate" if they are obtained according to the same reproducible experimental description and procedure.[7] A particular experimentally obtained value is said to be reproducible if there is a high degree of agreement between measurements or observations conducted on replicate specimens in different locations by different people—that is, if the experimental value is found to have a high precision.[8] Both of these are key features of reproducibility.
History
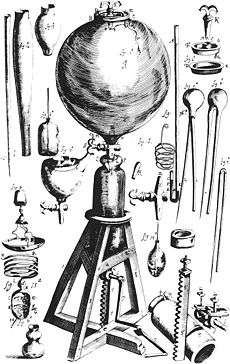
The first to stress the importance of reproducibility in science was the Irish chemist Robert Boyle, in England in the 17th century. Boyle's air pump was designed to generate and study vacuum, which at the time was a very controversial concept. Indeed, distinguished philosophers such as René Descartes and Thomas Hobbes denied the very possibility of vacuum existence. Historians of science Steven Shapin and Simon Schaffer, in their 1985 book Leviathan and the Air-Pump, describe the debate between Boyle and Hobbes, ostensibly over the nature of vacuum, as fundamentally an argument about how useful knowledge should be gained. Boyle, a pioneer of the experimental method, maintained that the foundations of knowledge should be constituted by experimentally produced facts, which can be made believable to a scientific community by their reproducibility. By repeating the same experiment over and over again, Boyle argued, the certainty of fact will emerge.
The air pump, which in the 17th century was a complicated and expensive apparatus to build, also led to one of the first documented disputes over the reproducibility of a particular scientific phenomenon. In the 1660s, the Dutch scientist Christiaan Huygens built his own air pump in Amsterdam, the first one outside the direct management of Boyle and his assistant at the time Robert Hooke. Huygens reported an effect he termed "anomalous suspension", in which water appeared to levitate in a glass jar inside his air pump (in fact suspended over an air bubble), but Boyle and Hooke could not replicate this phenomenon in their own pumps. As Shapin and Schaffer describe, “it became clear that unless the phenomenon could be produced in England with one of the two pumps available, then no one in England would accept the claims Huygens had made, or his competence in working the pump”. Huygens was finally invited to England in 1663, and under his personal guidance Hooke was able to replicate anomalous suspension of water. Following this Huygens was elected a Foreign Member of the Royal Society. However, Shapin and Schaffer also note that “the accomplishment of replication was dependent on contingent acts of judgment. One cannot write down a formula saying when replication was or was not achieved”.[9]
The philosopher of science Karl Popper noted briefly in his famous 1934 book The Logic of Scientific Discovery that “non-reproducible single occurrences are of no significance to science”.[10] The statistician Ronald Fisher wrote in his 1935 book The Design of Experiments, which set the foundations for the modern scientific practice of hypothesis testing and statistical significance, that “we may say that a phenomenon is experimentally demonstrable when we know how to conduct an experiment which will rarely fail to give us statistically significant results”.[11] Such assertions express a common dogma in modern science that reproducibility is a necessary condition (although not necessarily sufficient) for establishing a scientific fact, and in practice for establishing scientific authority in any field of knowledge. However, as noted above by Shapin and Schaffer, this dogma is not well-formulated quantitatively, such as statistical significance for instance, and therefore it is not explicitly established how many times must a fact be replicated to be considered reproducible.
Reproducible data
Reproducibility is one component of the precision of a measurement or test method. The other component is repeatability which is the degree of agreement of tests or measurements on replicate specimens by the same observer in the same laboratory. Both repeatability and reproducibility are usually reported as a standard deviation. A reproducibility limit is the value below which the difference between two test results obtained under reproducibility conditions may be expected to occur with a probability of approximately 0.95 (95%).[8]
Reproducibility is determined from controlled interlaboratory test programs or a measurement systems analysis.[12][13]
Although they are often confused, there is an important distinction between replicates and an independent repetition of an experiment. Replicates are performed within an experiment. They are not and cannot provide independent evidence of reproducibility. Rather they serve as an internal "check" on an experiment and should not be shown as part of the experimental results within a scientific publication. It is the independent repetition of an experiment that serves to underpin its reproducibility.[14]
Reproducible research
The term reproducible research refers to the idea that the ultimate product of academic research is the paper along with the laboratory notebooks [15][16] and full computational environment used to produce the results in the paper such as the code, data, etc. that can be used to reproduce the results and create new work based on the research.[17][18][19][20][21] Typical examples of reproducible research comprise compendia of data, code and text files, often organised around an R Markdown source document[22] or a Jupyter notebook.[23]
Psychology has seen a renewal of internal concerns about irreproducible results. Researchers showed in a 2006 study that, of 141 authors of a publication from the American Psychology Association (APA) empirical articles, 103 (73%) did not respond with their data over a six-month period.[24] In a follow up study published in 2015, it was found that 246 out of 394 contacted authors of papers in APA journals did not share their data upon request (62%).[25] In a 2012 paper, it was suggested that researchers should publish data along with their works, and a dataset was released alongside as a demonstration.[26] In 2017, an article published in Scientific Data suggested that this may not be sufficient and that the whole analysis context should be disclosed.[27] In 2015, psychology became the first discipline to conduct and publish an open, registered empirical study of reproducibility called the Reproducibility Project. A group of 270 researchers from around the world collaborated to replicate 100 empirical studies from three top psychology journals. Fewer than half of the attempted replications were successful.[28]
There have been initiatives to improve reporting and hence reproducibility in the medical literature for many years, which began with the CONSORT initiative, which is now part of a wider initiative, the EQUATOR Network. This group has recently turned its attention to how better reporting might reduce waste in research,[29] especially biomedical research.
Reproducible research is key to new discoveries in pharmacology. A Phase I discovery will be followed by Phase II reproductions as a drug develops towards commercial production. In recent decades Phase II success has fallen from 28% to 18%. A 2011 study found that 65% of medical studies were inconsistent when re-tested, and only 6% were completely reproducible.[30]
In 2012, a study by Begley and Ellis was published in Nature that reviewed a decade of research. That study found that 47 out of 53 medical research papers focused on cancer research were irreproducible.[31] The irreproducible studies had a number of features in common, including that studies were not performed by investigators blinded to the experimental versus the control arms, there was a failure to repeat experiments, a lack of positive and negative controls, failure to show all the data, inappropriate use of statistical tests and use of reagents that were not appropriately validated.[32] John P. A. Ioannidis writes, "While currently there is unilateral emphasis on 'first' discoveries, there should be as much emphasis on replication of discoveries."[33] The Nature study was itself reproduced in the journal PLOS ONE, which confirmed that a majority of cancer researchers surveyed had been unable to reproduce a result.[34]
In 2016, Nature conducted a survey of 1,576 researchers who took a brief online questionnaire on reproducibility in research. According to the survey, more than 70% of researchers have tried and failed to reproduce another scientist's experiments, and more than half have failed to reproduce their own experiments. "Although 52% of those surveyed agree there is a significant 'crisis' of reproducibility, less than 31% think failure to reproduce published results means the result is probably wrong, and most say they still trust the published literature."[35]
A 2018 study published in the journal PLOS ONE found that 14.4% of a sample of public health researchers had shared their data or code or both[36] and a related study found limited reproducibility in six public health studies.[37] Data and statistical code sharing, along with following statistical coding guidelines, are recommended practices for improving reproducibility.[38]
Noteworthy irreproducible results
Hideyo Noguchi became famous for correctly identifying the bacterial agent of syphilis, but also claimed that he could culture this agent in his laboratory. Nobody else has been able to produce this latter result.[39]
In March 1989, University of Utah chemists Stanley Pons and Martin Fleischmann reported the production of excess heat that could only be explained by a nuclear process ("cold fusion"). The report was astounding given the simplicity of the equipment: it was essentially an electrolysis cell containing heavy water and a palladium cathode which rapidly absorbed the deuterium produced during electrolysis. The news media reported on the experiments widely, and it was a front-page item on many newspapers around the world (see science by press conference). Over the next several months others tried to replicate the experiment, but were unsuccessful.[40]
Nikola Tesla claimed as early as 1899 to have used a high frequency current to light gas-filled lamps from over 25 miles (40 km) away without using wires. In 1904 he built Wardenclyffe Tower on Long Island to demonstrate means to send and receive power without connecting wires. The facility was never fully operational and was not completed due to economic problems, so no attempt to reproduce his first result was ever carried out.[41]
Other examples which contrary evidence has refuted the original claim:
- Stimulus-triggered acquisition of pluripotency, revealed to be the result of fraud
- GFAJ-1, a bacterium that could purportedly incorporate arsenic into its DNA in place of phosphorus
- MMR vaccine controversy – a study in The Lancet claiming the MMR vaccine caused autism was revealed to be fraudulent
- Schön scandal – semiconductor "breakthroughs" revealed to be fraudulent
- Power posing – a social psychology phenomenon that went viral after being the subject of a very popular TED talk, but was unable to be replicated in dozens of studies[42]
Stochastic processes
The reproducibility requirement cannot be applied to individual samples of phenomena which have a partially or totally non-deterministic nature. However, it still applies to the probabilistic description of such phenomena, with error tolerance given by probability theory.
See also
References
- McCullough, B. D. & McGeary, Kerry Anne & Harrison, Teresa D. (2006), "Lessons from the JMCB Archive", Journal of Money, Credit and Banking (in German), 38 (4), pp. 1093–1107, doi:10.1353/mcb.2006.0061CS1 maint: multiple names: authors list (link)
- "JCGM 100:2008. Evaluation of measurement data – Guide to the expression of uncertainty in measurement" (PDF). Joint Committee for Guides in Metrology. 2008. Retrieved 7 August 2020.
- Taylor, Barry N.; Kuyatt, Chris E. (1994), NIST Guidelines for Evaluating and Expressing the Uncertainty of NIST Measurement Results Cover, Gaithersburg, MD, US: National Institute of Standards and Technology
- Leek, Jeffrey T; Peng, Roger D (February 10, 2015). "Reproducible research can still be wrong: Adopting a prevention approach". Proceedings of the National Academy of Sciences of the United States of America. 112 (6): 1645–1646. arXiv:1502.03169. Bibcode:2015PNAS..112.1645L. doi:10.1073/pnas.1421412111. PMC 4330755. PMID 25670866.
- Repko, Allen F.; Szostak, Rick (2007) [1959]. Interdisciplinary research: process and theory (Third ed.). Los Angeles. ISBN 9781506330488. OCLC 936687178.
Replicability and reproducibility, after all, are central to the creation and testing of theories and their acceptance by scientific communities. (Berg, 2004, p. 7).
- Munafò, Marcus (29 March 2017). "Metascience: Reproducibility blues". Nature. 543 (7647): 619–620. Bibcode:2017Natur.543..619M. doi:10.1038/543619a.
- "Definition of Commensurate". Merriam-Webster. 2019-01-09. Retrieved 2019-01-09.
- Subcommittee E11.20 on Test Method Evaluation and Quality Control (2014). "Standard Practice for Use of the Terms Precision and Bias in ASTM Test Methods". ASTM International. ASTM E177.(subscription required)
- Steven Shapin and Simon Schaffer, Leviathan and the Air-Pump, Princeton University Press, Princeton, New Jersey (1985).
- This citation is from the 1959 translation to English, Karl Popper, The Logic of Scientific Discovery, Routledge, London, 1992, p. 66.
- Ronald Fisher, The Design of Experiments, (1971) [1935](9th ed.), Macmillan, p. 14.
- ASTM E691 Standard Practice for Conducting an Interlaboratory Study to Determine the Precision of a Test Method
- ASTM F1469 Standard Guide for Conducting a Repeatability and Reproducibility Study on Test Equipment for Nondestructive Testing
- Vaux, D. L.; Fidler, F.; Cumming, G. (2012). "Replicates and repeats—what is the difference and is it significant?: A brief discussion of statistics and experimental design". EMBO Reports. 13 (4): 291–296. doi:10.1038/embor.2012.36. PMC 3321166. PMID 22421999.
- King, Gary (1995). "Replication, Replication". PS: Political Science and Politics. 28 (3): 444–452. doi:10.2307/420301. ISSN 1049-0965. JSTOR 420301.
- Kühne, Martin; Liehr, Andreas W. (2009). "Improving the Traditional Information Management in Natural Sciences". Data Science Journal. 8 (1): 18–27. doi:10.2481/dsj.8.18.
- Fomel, Sergey; Claerbout, Jon (2009). "Guest Editors' Introduction: Reproducible Research". Computing in Science and Engineering. 11 (1): 5–7. Bibcode:2009CSE....11a...5F. doi:10.1109/MCSE.2009.14.
- Buckheit, Jonathan B.; Donoho, David L. (May 1995). WaveLab and Reproducible Research (PDF) (Report). California, United States: Stanford University, Department of Statistics. Technical Report No. 474. Retrieved 5 January 2015.
- "The Yale Law School Round Table on Data and Core Sharing: "Reproducible Research"". Computing in Science and Engineering. 12 (5): 8–12. 2010. doi:10.1109/MCSE.2010.113.
- Marwick, Ben (2016). "Computational reproducibility in archaeological research: Basic principles and a case study of their implementation". Journal of Archaeological Method and Theory. 24 (2): 424–450. doi:10.1007/s10816-015-9272-9.
- Goodman, Steven N.; Fanelli, Daniele; Ioannidis, John P. A. (1 June 2016). "What does research reproducibility mean?". Science Translational Medicine. 8 (341): 341ps12. doi:10.1126/scitranslmed.aaf5027. PMID 27252173.
- Marwick, Ben; Boettiger, Carl; Mullen, Lincoln (29 September 2017). "Packaging data analytical work reproducibly using R (and friends)". The American Statistician. 72: 80–88. doi:10.1080/00031305.2017.1375986.
- Kluyver, Thomas; Ragan-Kelley, Benjamin; Perez, Fernando; Granger, Brian; Bussonnier, Matthias; Frederic, Jonathan; Kelley, Kyle; Hamrick, Jessica; Grout, Jason; Corlay, Sylvain (2016). "Jupyter Notebooks-a publishing format for reproducible computational workflows" (PDF). In Loizides, F; Schmidt, B (eds.). Positioning and Power in Academic Publishing: Players, Agents and Agendas. IOS Press. pp. 87–90.
- Wicherts, J. M.; Borsboom, D.; Kats, J.; Molenaar, D. (2006). "The poor availability of psychological research data for reanalysis". American Psychologist. 61 (7): 726–728. doi:10.1037/0003-066X.61.7.726. PMID 17032082.
- Vanpaemel, W.; Vermorgen, M.; Deriemaecker, L.; Storms, G. (2015). "Are we wasting a good crisis? The availability of psychological research data after the storm". Collabra. 1 (1): 1–5. doi:10.1525/collabra.13.
- Wicherts, J. M.; Bakker, M. (2012). "Publish (your data) or (let the data) perish! Why not publish your data too?". Intelligence. 40 (2): 73–76. doi:10.1016/j.intell.2012.01.004.
- Pasquier, Thomas; Lau, Matthew K.; Trisovic, Ana; Boose, Emery R.; Couturier, Ben; Crosas, Mercè; Ellison, Aaron M.; Gibson, Valerie; Jones, Chris R.; Seltzer, Margo (5 September 2017). "If these data could talk". Scientific Data. 4: 170114. Bibcode:2017NatSD...470114P. doi:10.1038/sdata.2017.114. PMC 5584398. PMID 28872630.
- Open Science Collaboration (2015). "Estimating the reproducibility of Psychological Science" (PDF). Science. 349 (6251): aac4716. doi:10.1126/science.aac4716. hdl:10722/230596. PMID 26315443.
- "Research Waste/EQUATOR Conference | Research Waste". researchwaste.net. Archived from the original on 29 October 2016.
- Prinz, F.; Schlange, T.; Asadullah, K. (2011). "Believe it or not: How much can we rely on published data on potential drug targets?". Nature Reviews Drug Discovery. 10 (9): 712. doi:10.1038/nrd3439-c1. PMID 21892149.
- Begley, C. G.; Ellis, L. M. (2012). "Drug development: Raise standards for preclinical cancer research". Nature. 483 (7391): 531–533. Bibcode:2012Natur.483..531B. doi:10.1038/483531a. PMID 22460880 – via Semantic Scholar.
- Begley, CG (2013). "Reproducibility: six flags for suspect work". Nature. 497 (7450): 433–434. Bibcode:2013Natur.497..433B. doi:10.1038/497433a. PMID 23698428.
- Is the spirit of Piltdown man alive and well?
- Mobley, A.; Linder, S. K.; Braeuer, R.; Ellis, L. M.; Zwelling, L. (2013). Arakawa, Hirofumi (ed.). "A Survey on Data Reproducibility in Cancer Research Provides Insights into Our Limited Ability to Translate Findings from the Laboratory to the Clinic". PLOS ONE. 8 (5): e63221. Bibcode:2013PLoSO...863221M. doi:10.1371/journal.pone.0063221. PMC 3655010. PMID 23691000.
- Baker, Monya (2016). "1,500 scientists lift the lid on reproducibility". Nature. 533 (7604): 452–454. Bibcode:2016Natur.533..452B. doi:10.1038/533452a. PMID 27225100.
- Harris, Jenine K.; Johnson, Kimberly J.; Carothers, Bobbi J.; Combs, Todd B.; Luke, Douglas A.; Wang, Xiaoyan (2018). "Use of reproducible research practices in public health: A survey of public health analysts". PLOS ONE. 13 (9): e0202447. Bibcode:2018PLoSO..1302447H. doi:10.1371/journal.pone.0202447. ISSN 1932-6203. OCLC 7891624396. PMC 6135378. PMID 30208041.
- Harris, Jenine K; Wondmeneh, Sarah B; Zhao, Yiqiang; Leider, Jonathon P (2019). "Examining the Reproducibility of 6 Published Studies in Public Health Services and Systems Research". Journal of Public Health Management and Practice Journal of Public Health Management and Practice. 25 (2): 128–136. doi:10.1097/PHH.0000000000000694. ISSN 1078-4659. OCLC 7338887045. PMID 29481544.
- Harris J.K; Johnson K.J; Combs T.B; Carothers B.J; Luke D.A; Wang X (2019). "Three Changes Public Health Scientists Can Make to Help Build a Culture of Reproducible Research". Public Health Rep. Public Health Reports. 134 (2): 109–111. doi:10.1177/0033354918821076. ISSN 0033-3549. OCLC 7991854250. PMC 6410469. PMID 30657732.
- Tan, SY; Furubayashi, J (2014). "Hideyo Noguchi (1876-1928): Distinguished bacteriologist". Singapore Medical Journal. 55 (10): 550–551. doi:10.11622/smedj.2014140. ISSN 0037-5675. PMC 4293967. PMID 25631898.
- Browne, Malcolm (3 May 1989). "Physicists Debunk Claim Of a New Kind of Fusion". New York Times. Retrieved 3 February 2017.
- Cheney, Margaret (1999), Tesla, Master of Lightning, New York: Barnes & Noble Books, ISBN 0-7607-1005-8, pp. 107.; "Unable to overcome his financial burdens, he was forced to close the laboratory in 1905."
- Dominus, Susan (October 18, 2017). "When the Revolution Came for Amy Cuddy". New York Times Magazine.
- Turner, William (1903), History of Philosophy, Ginn and Company, Boston, MA, Etext. See especially: "Aristotle".
- Definition, by International Union of Pure and Applied Chemistry
Further reading
- Blow, Nathan S. (January 2014). "A Simple Question of Reproducibility". From the Editor. BioTechniques. 16 (1): 8. doi:10.2144/000114117. PMID 24592483.
It is interesting to note that at this moment of greater irreproducibility in life science, journals continue to minimize the space given to Materials and Methods sections in articles.
- Timmer, John (October 2006). "Scientists on Science: Reproducibility". Arstechnica.
- Saey, Tina Hesman (January 2015). "Is redoing scientific research the best way to find truth? During replication attempts, too many studies fail to pass muster". Science News. "Science is not irrevocably broken, [epidemiologist John Ioannidis] asserts. It just needs some improvements. "Despite the fact that I’ve published papers with pretty depressive titles, I’m actually an optimist,” Ioannidis says. “I find no other investment of a society that is better placed than science.”"
External links
![]() |
Look up reproducibility in Wiktionary, the free dictionary. |
- Reproducible papers with artifacts
- Guidelines for Evaluating and Expressing the Uncertainty of NIST Measurement Results
- Definition of reproducibility in the IUPAC Gold Book
- ReproducibleResearch.net
- cTuning.org - reproducible research and experimentation in computer engineering
- Transparency and Openness Promotion (TOP) Guidelines
- Center for Open Science TOP Guidelines