Gene expression profiling in cancer
Cancer is a category of disease characterized by uncontrolled cell growth and proliferation. For cancer to develop, genes regulating cell growth and differentiation must be altered; these mutations are then maintained through subsequent cell divisions and are thus present in all cancerous cells. Gene expression profiling is a technique used in molecular biology to query the expression of thousands of genes simultaneously. In the context of cancer, gene expression profiling has been used to more accurately classify tumors. The information derived from gene expression profiling often helps in predicting the patient’s clinical outcome.
Background
Oncogenesis
Oncogenesis is the process by which normal cells acquire the properties of cancer cells leading to the formation of a cancer or tumor (see: tumorigenesis). It is characterized by a molecular reprogramming of a cell to undergo uninhibited cell division, allowing the formation of a malignant mass. The cells forming this mass undergo natural selection: as cells acquire mutations that enhance their survivability or reproductive capacity, they dominate the growing tumor as other cells are out-competed (see: somatic evolution in cancer). Because of these selective properties, the majority of cells within a tumor will share a common profile of gene expression.
Gene expression profiling
Gene expression profiling is a technique used in molecular biology to query the expression of thousands of genes simultaneously. While almost all cells in an organism contain the entire genome of the organism, only a small subset of those genes is expressed as messenger RNA (mRNA) at any given time, and their relative expression can be evaluated. Techniques include DNA microarray technology or sequenced-based techniques such as serial analysis of gene expression (SAGE).
Current cancer research makes use primarily of DNA microarrays in which an arrayed series of microscopic spots of pre-defined DNA oligonucleotides known as probes are covalently attached to a solid surface such as glass, forming what is known as a gene chip. DNA labeled with fluorophores (target) is prepared from a sample such as a tumor biopsy and is hybridized to the complementary DNA (cDNA) sequences on the gene chip. The chip is then scanned for the presence and strength of the fluorescent labels at each spot representing probe-target hybrids. The level of fluorescence at a particular spot provides quantitative information about the expression of the particular gene corresponding to the spotted cDNA sequence. DNA microarrays evolved from Southern blotting which allows for detection of a specific DNA sequence in a sample of DNA.
Due to lowering costs, RNA-Sequencing is becoming more common as a method for cancer gene expression profiling. It is superior to microarray techniques due to not having the bias inherent in probe selection.
Cancer classification
Background
.jpg)
Classification of cancers has been dominated by the fields of histology and histopathology which aim to leverage morphological markers for accurate identification of a tumor type. Histological methods rely on chemical staining of tissues with pigments such as haematoxylin and eosin and microscopy-based visualization by a pathologist. The identification of tumor subtypes is based on established classification schemes such as the International Classification of Diseases published by the World Health Organization which provides codes to classify diseases and a wide variety of signs, symptoms, abnormal findings, complaints, social circumstances, and external causes of injury or diseases. For some types of cancer, these methods are unable to distinguish between subclasses; for example, defining subgroups of diffuse large B-cell lymphoma (DLBCL) have largely failed due to discrepancies between inter- and intra-observer reproducibility.[1] Furthermore, the clinical outcomes of tumors classified as DLBCLs is highly variable[1] suggesting that there are multiple subtypes of DLBCL that cannot be distinguished based on these histological markers. Breast tumor classification too has largely failed based on these predictors.[2] Development of effective therapies depends on accurate diagnosis; additionally, poor diagnosis can lead to patient suffering due to needless side-effects from non-targeted treatments and to increased health care expenditure. Most telling perhaps is that 70-80% of breast cancer patients receiving chemotherapy based on traditional predictors would have survived without it.[3][4]
Of note, similar gene expression patterns associated with metastatic behaviour of breast cancer tumor cells have also been found in breast cancer of dog, the most common tumor of the female dog.[5][6]
Presented below are ways that gene expression profiling has been used to more precisely classify tumors into subgroups, often with clinical effect.
Molecular Signature
In a particular type of cell or tissue, only a small subset of an organism’s genomic DNA will be expressed as mRNAs at any given time. The unique pattern of gene expression for a given cell or tissue is referred to as its molecular signature. For example, the expression of genes in skin cells would be very different compared to those expressed in blood cells. Microarray analysis can provide quantitative gene expression information allowing for the generation of a molecular signature, each unique to a particular class of tumor. This idea was first shown experimentally[7] in 2000 by researchers at Stanford University published in Nature Genetics. The authors measured the relative expression of 9,703 human cDNAs in sixty cancer cell lines previously studied and characterized by the National Cancer Institute’s Developmental Therapeutics Program. A hierarchical clustering algorithm was used to group cell lines based on the similarity by which the pattern of gene expression varied. In this study by Ross et al., the majority of cell lines with common organs of origin (based on information from the National Institutes of Health) clustered together at terminal branches, suggesting that cancer cells arising from the same tissue share many molecular characteristics. This allows for reliable identification of tumor type based on gene expression.
Tumor subclass
A more powerful result of gene expression profiling is the ability to further classify tumors into subtypes having distinct biological properties and affect prognoses. For example, some diffuse large B-cell lymphomas (DLBCLs) are indistinguishable based on histological methods yet are clinically heterogeneous: 40% of patients respond well and exhibit prolonged survival while the remaining 60% do not.[8]
In 2000, Stanford researchers led by Ash Alizadeh and colleagues published results[8] in Nature, utilizing expression profiling techniques to stratify DLBCL to two subtypes: germinal center B-like DLBCL and activated B-like DLBCL. The authors developed custom microarrays termed “lymphochips” that were used to query expression of 17,856 genes preferentially expressed in lymphoid cells and those with roles in cancer or immunology for 96 lymphocyte samples. The hierarchical clustering algorithm identified a subset of tumors that would have been labeled DLBCLs by traditional histological methods; however, the expression profiles of these tumors were heterogeneous. When the tumors were re-clustered based on expression of germinal center B-cell genes, a second group of genes characteristic of activated B-cells emerged and were oppositely regulated compared to the first set of genes. Based on these expression patterns, the heterogeneous DLBCL cluster was subclassified to the germinal center B-like DLBCL and activated B-like DLBCL. The distinction of these groups is significant in terms of patient overall survival: the probability of survival for patients with germinal center B-like DLBCLs over 10 years was about 80% while those with activated B-like DLBCLs was lowered to about 40% over a shorter eight-year period.
Breast cancers are also difficult to distinguish based on histological markers. In a 2000 study published in Nature, Stanford researchers led by Perou, C.M. characterized gene expression patterns across 8,102 genes for 65 biopsies obtained from breast cancers.[9] The goal of the study was to identify patterns of gene expression that could be used to describe the phenotypic diversity of breast tumors by comparing the profiles of the biopsies to those of cultured cell lines and relating this information to clinical data. The tumors were clustered into two major groups that largely reflected the ER-positive and ER-negative clinical descriptions. The ER-positive tumors were characterized by high expression of genes normally expressed in breast luminal cells. The authors suggest that this higher-order distinction may encompass at least two biologically distinct types of cancer that may each require a unique course of treatment. Within the ER-negative group, additional clusters were identified based on expression of Erb-B2 and keratin 5- and 17-enriched basal epithelial-like genes. These groups reflect distinct molecular features as related to mammary epithelial biology, based on the outcome of disease.
Clinical application
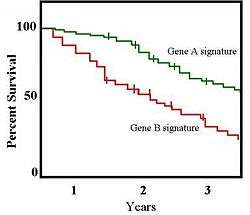
In a 2001 study published in the Proceedings of the National Academy of Sciences, Sørlie et al.[10] further stratified the classifications described by Perou et al.[9] and explored the clinical value of these breast cancer subtypes. The authors separated the ER-positive tumors into two distinct groups and found that tumor classification based on gene expression was related to patient survival. The expression of 427 genes was measured for 78 cancers and seven non-malignant breast samples. Following hierarchical clustering, the samples formed two groups at the highest level of organization reflecting the ER-positive and ER-negative phenotypes; the ER-negative cluster further stratified to groups identical to those described by Perou et al.[9] In contrast to previous results, Sørlie et al.[10] found that the ER-positive group could also be separated into three distinct subgroups termed luminal subtypes A, B, and, C based on patterns of luminal-specific gene expression with different outcomes. The authors further found once they performed survival analyses that tumors belonging to the various groups showed significantly different outcomes when treated uniformly. Survival analyses are often shown as Kaplan-Meier survival plots, an example of which is shown to the right.
In addition to identifying genes that correlate to survival, microarray analyses have been utilized to establish gene expression profiles associated with prognosis. It is agreed upon that patients with tumors exhibiting poor prognostic features would benefit the most from adjuvant therapy as these treatments substantially improve overall survival for women with breast cancer. Traditional prognostic factors, however, are inexact as mentioned above. Researchers at the Netherlands Cancer Institute were able to identify "good-prognosis" and "bad-prognosis" signatures based on the expression of 70 genes that was better able to predict the likelihood of metastasis development within five years for breast cancer patients[11][12] Metastasis involves the spread of cancer from one organ to others throughout the body and is the principal cause of death in cancer patients. While the study at the Netherlands Cancer Institute applied to breast cancer patients only, researchers at Massachusetts Institute of Technology identified a molecular signature of metastasis that applied to adenocarcinomas in general.[13]
References
- "The Non-Hodgkin's Lymphoma Classification Project: A clinical evaluation of the International Lymphoma Study Group classification of non-Hodgkin's lymphoma". Blood. 89 (11): 3909–3918. 1997. doi:10.1182/blood.V89.11.3909. PMID 9166827.
- McGuire W.L. (1991). "Breast cancer prognostic factors: evaluation guidelines". Journal of the National Cancer Institute. 83 (3): 154–5. doi:10.1093/jnci/83.3.154. PMID 1988696.
- Early Breast Cancer Trialists' Collaborative Group (1998). "Polychemotherapy for early breast cancer: an overview of the randomised trials". The Lancet. 352 (9132): 930–942. doi:10.1016/S0140-6736(98)03301-7.
- Foo X (1998). "Tamoxifen for early breast cancer: an overview of the randomised trials". The Lancet. 351 (9114): 1451–1467. doi:10.1016/S0140-6736(97)11423-4. PMID 9605801.
- Klopfleisch R, Lenze D, Hummel M, Gruber AD (2010). "Metastatic canine mammary carcinomas can be identified by a gene expression profile that partly overlaps with human breast cancer profiles". BMC Cancer. 10: 618. doi:10.1186/1471-2407-10-618. PMC 2994823. PMID 21062462.
- Klopfleisch R, Lenze D, Hummel M, Gruber AD (2010). "The metastatic cascade is reflected in the transcriptome of metastatic canine mammary carcinomas". Veterinary Journal. 190 (2): 236–243. doi:10.1016/j.tvjl.2010.10.018. PMID 21112801.
- Ross D.T.; et al. (2000). "Systematic variation in gene expression patterns in human cancer cell lines". Nature Genetics. 24 (3): 227–235. doi:10.1038/73432. PMID 10700174.
- Alizadeh A.A.; et al. (2000). "Distinct types of diffuse large B-cell lymphoma identified by gene expression profiling". Nature. 403 (6769): 503–511. doi:10.1038/35000501. PMID 10676951.
- Perou C.M.; et al. (2000). "Molecular portraits of human breast tumors". Nature. 406 (6797): 747–752. doi:10.1038/35021093. PMID 10963602.
- Sørlie M, et al. (2001). "Gene expression patterns of breast carcinomas distinguish tumor subclasses with clinical implications". Proceedings of the National Academy of Sciences. 98 (19): 10869–10874. doi:10.1073/pnas.191367098. PMC 58566. PMID 11553815.
- van't Veer L.J.; et al. (2002). "Gene expression profiling predicts clinical outcome of breast cancer". Nature. 415 (6871): 530–536. doi:10.1038/415530a. hdl:1874/15552. PMID 11823860.
- van de Vijver M.J.; et al. (2002). "A gene-expression signature as a predictor of survival in breast cancer". The New England Journal of Medicine. 347 (25): 1999–2009. doi:10.1056/NEJMoa021967. hdl:1874/15577. PMID 12490681.
- Ramaswamy S, et al. (2002). "A molecular signature of metastasis in primary solid tumors". Nature Genetics. 33 (1): 49–54. doi:10.1038/ng1060. PMID 12469122.
- Gibson, Greg; Muse, Spencer V. (2009). A Primer of Genome Science. Sunderland, Massachusetts: Sinauer Associates, Inc. ISBN 978-0-87893-236-8.
- Weinberg, Robert A. (2007). The Biology of Cancer. New York: Garland Science, Taylor & Francis Group, LLC. ISBN 978-0-8153-4076-8.
Resources
- The Genevestigator Neoplasm tool is a free and open access tool to visualize the expression of genes across more than 1,000 different cancer types and subtypes.