Eye tracking
Eye tracking is the process of measuring either the point of gaze (where one is looking) or the motion of an eye relative to the head. An eye tracker is a device for measuring eye positions and eye movement. Eye trackers are used in research on the visual system, in psychology, in psycholinguistics, marketing, as an input device for human-computer interaction, and in product design. Eye trackers are also being increasingly used for rehabilitative and assistive applications (related for instance to control of wheel chairs, robotic arms and prostheses). There are a number of methods for measuring eye movement. The most popular variant uses video images from which the eye position is extracted. Other methods use search coils or are based on the electrooculogram.
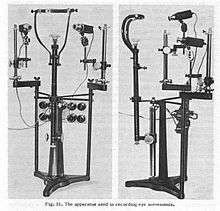
History
In the 1800s, studies of eye movement were made using direct observations.
In 1879 in Paris, Louis Émile Javal observed that reading does not involve a smooth sweeping of the eyes along the text, as previously assumed, but a series of short stops (called fixations) and quick saccades.[1] This observation raised important questions about reading, questions which were explored during the 1900s: On which words do the eyes stop? For how long? When do they regress to already seen words?
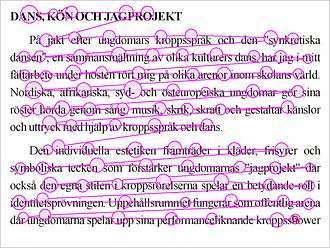
Edmund Huey[2] built an early eye tracker, using a sort of contact lens with a hole for the pupil. The lens was connected to an aluminum pointer that moved in response to the movement of the eye. Huey studied and quantified regressions (only a small proportion of saccades are regressions), and he showed that some words in a sentence are not fixated.
The first non-intrusive eye-trackers were built by Guy Thomas Buswell in Chicago, using beams of light that were reflected on the eye and then recording them on film. Buswell made systematic studies into reading[3] and picture viewing.[4]
In the 1950s, Alfred L. Yarbus[5] did important eye tracking research and his 1967 book is often quoted. He showed that the task given to a subject has a very large influence on the subject's eye movement. He also wrote about the relation between fixations and interest:
- "All the records ... show conclusively that the character of the eye movement is either completely independent of or only very slightly dependent on the material of the picture and how it was made, provided that it is flat or nearly flat."[6] The cyclical pattern in the examination of pictures "is dependent on not only what is shown on the picture, but also the problem facing the observer and the information that he hopes to gain from the picture."[7]
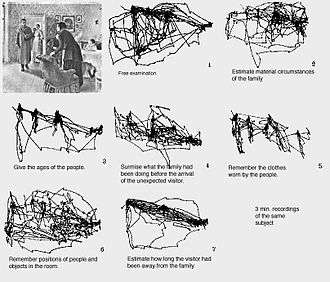
- "Records of eye movements show that the observer's attention is usually held only by certain elements of the picture.... Eye movement reflects the human thought processes; so the observer's thought may be followed to some extent from records of eye movement (the thought accompanying the examination of the particular object). It is easy to determine from these records which elements attract the observer's eye (and, consequently, his thought), in what order, and how often."[6]
- "The observer's attention is frequently drawn to elements which do not give important information but which, in his opinion, may do so. Often an observer will focus his attention on elements that are unusual in the particular circumstances, unfamiliar, incomprehensible, and so on."[8]
- "... when changing its points of fixation, the observer's eye repeatedly returns to the same elements of the picture. Additional time spent on perception is not used to examine the secondary elements, but to reexamine the most important elements."[9]
In the 1970s, eye-tracking research expanded rapidly, particularly reading research. A good overview of the research in this period is given by Rayner.[13]
In 1980, Just and Carpenter[14] formulated the influential Strong eye-mind hypothesis, that "there is no appreciable lag between what is fixated and what is processed". If this hypothesis is correct, then when a subject looks at a word or object, he or she also thinks about it (process cognitively), and for exactly as long as the recorded fixation. The hypothesis is often taken for granted by researchers using eye-tracking. However, gaze-contingent techniques offer an interesting option in order to disentangle overt and covert attentions, to differentiate what is fixated and what is processed.
During the 1980s, the eye-mind hypothesis was often questioned in light of covert attention,[15][16] the attention to something that one is not looking at, which people often do. If covert attention is common during eye-tracking recordings, the resulting scan-path and fixation patterns would often show not where our attention has been, but only where the eye has been looking, failing to indicate cognitive processing.
The 1980s also saw the birth of using eye-tracking to answer questions related to human-computer interaction. Specifically, researchers investigated how users search for commands in computer menus.[17] Additionally, computers allowed researchers to use eye-tracking results in real time, primarily to help disabled users.[17]
More recently, there has been growth in using eye tracking to study how users interact with different computer interfaces. Specific questions researchers ask are related to how easy different interfaces are for users.[17] The results of the eye tracking research can lead to changes in design of the interface. Yet another recent area of research focuses on Web development. This can include how users react to drop-down menus or where they focus their attention on a website so the developer knows where to place an advertisement.[18]
According to Hoffman,[19] current consensus is that visual attention is always slightly (100 to 250 ms) ahead of the eye. But as soon as attention moves to a new position, the eyes will want to follow.[20]
We still cannot infer specific cognitive processes directly from a fixation on a particular object in a scene.[21] For instance, a fixation on a face in a picture may indicate recognition, liking, dislike, puzzlement etc. Therefore, eye tracking is often coupled with other methodologies, such as introspective verbal protocols.
Thanks to advancement in portable electronic devices, portable head-mounted eye trackers currently can achieve excellent performance and are being increasingly used in research and market applications targeting daily life settings.[22] These same advances have led to increases in the study of small eye movements that occur during fixation, both in the lab and in applied settings.[23]
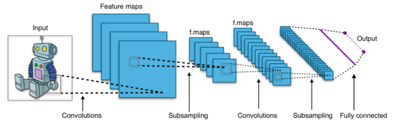
In the 21st century, the use of artificial intelligence (AI) and artificial neural networks has become a viable way to complete eye-tracking tasks and analysis. In particular, the convolutional neural network lends itself to eye-tracking, as it is designed for image-centric tasks. With AI, eye-tracking tasks and studies can yield additional information that may not have been detected by human observers. The practice of deep learning also allows for a given neural network to improve at a given task when given enough sample data. This requires a relatively large supply of training data, however.[24]
The potential use cases for AI in eye-tracking cover a wide range of topics from medical applications[25] to driver safety[24] to game theory.[26] While the CNN structure may fit relatively well with the task of eye-tracking, researchers have the option to construct a custom neural network that is tailored for the specific task at hand. In those instances, these in-house creations can outperform pre-existing templates for a neural network.[27] In this sense, it remains to be seen if there is a way to determine the ideal network structure for a given task.
Tracker types
Eye-trackers measure rotations of the eye in one of several ways, but principally they fall into one of three categories: (i) measurement of the movement of an object (normally, a special contact lens) attached to the eye; (ii) optical tracking without direct contact to the eye; and (iii) measurement of electric potentials using electrodes placed around the eyes.
Eye-attached tracking
The first type uses an attachment to the eye, such as a special contact lens with an embedded mirror or magnetic field sensor, and the movement of the attachment is measured with the assumption that it does not slip significantly as the eye rotates. Measurements with tight-fitting contact lenses have provided extremely sensitive recordings of eye movement, and magnetic search coils are the method of choice for researchers studying the dynamics and underlying physiology of eye movement. This method allows the measurement of eye movement in horizontal, vertical and torsion directions.[28]
Optical tracking
The second broad category uses some non-contact, optical method for measuring eye motion. Light, typically infrared, is reflected from the eye and sensed by a video camera or some other specially designed optical sensor. The information is then analyzed to extract eye rotation from changes in reflections. Video-based eye trackers typically use the corneal reflection (the first Purkinje image) and the center of the pupil as features to track over time. A more sensitive type of eye-tracker, the dual-Purkinje eye tracker,[29] uses reflections from the front of the cornea (first Purkinje image) and the back of the lens (fourth Purkinje image) as features to track. A still more sensitive method of tracking is to image features from inside the eye, such as the retinal blood vessels, and follow these features as the eye rotates. Optical methods, particularly those based on video recording, are widely used for gaze-tracking and are favored for being non-invasive and inexpensive.
Electric potential measurement
The third category uses electric potentials measured with electrodes placed around the eyes. The eyes are the origin of a steady electric potential field which can also be detected in total darkness and if the eyes are closed. It can be modelled to be generated by a dipole with its positive pole at the cornea and its negative pole at the retina. The electric signal that can be derived using two pairs of contact electrodes placed on the skin around one eye is called Electrooculogram (EOG). If the eyes move from the centre position towards the periphery, the retina approaches one electrode while the cornea approaches the opposing one. This change in the orientation of the dipole and consequently the electric potential field results in a change in the measured EOG signal. Inversely, by analysing these changes in eye movement can be tracked. Due to the discretisation given by the common electrode setup, two separate movement components – a horizontal and a vertical – can be identified. A third EOG component is the radial EOG channel,[30] which is the average of the EOG channels referenced to some posterior scalp electrode. This radial EOG channel is sensitive to the saccadic spike potentials stemming from the extra-ocular muscles at the onset of saccades, and allows reliable detection of even miniature saccades.[31]
Due to potential drifts and variable relations between the EOG signal amplitudes and the saccade sizes, it is challenging to use EOG for measuring slow eye movement and detecting gaze direction. EOG is, however, a very robust technique for measuring saccadic eye movement associated with gaze shifts and detecting blinks. Contrary to video-based eye-trackers, EOG allows recording of eye movements even with eyes closed, and can thus be used in sleep research. It is a very light-weight approach that, in contrast to current video-based eye-trackers, requires only very low computational power; works under different lighting conditions; and can be implemented as an embedded, self-contained wearable system.[32][33] It is thus the method of choice for measuring eye movement in mobile daily-life situations and REM phases during sleep. The major disadvantage of EOG is its relatively poor gaze-direction accuracy compared to a video tracker. That is, it is difficult to determine with good accuracy exactly where a subject is looking, though the time of eye movements can be determined.
Technologies and techniques
The most widely used current designs are video-based eye-trackers. A camera focuses on one or both eyes and records eye movement as the viewer looks at some kind of stimulus. Most modern eye-trackers use the center of the pupil and infrared / near-infrared non-collimated light to create corneal reflections (CR). The vector between the pupil center and the corneal reflections can be used to compute the point of regard on surface or the gaze direction. A simple calibration procedure of the individual is usually needed before using the eye tracker.[34]
Two general types of infrared / near-infrared (also known as active light) eye-tracking techniques are used: bright-pupil and dark-pupil. Their difference is based on the location of the illumination source with respect to the optics. If the illumination is coaxial with the optical path, then the eye acts as a retroreflector as the light reflects off the retina creating a bright pupil effect similar to red eye. If the illumination source is offset from the optical path, then the pupil appears dark because the retroreflection from the retina is directed away from the camera.[35]
Bright-pupil tracking creates greater iris/pupil contrast, allowing more robust eye-tracking with all iris pigmentation, and greatly reduces interference caused by eyelashes and other obscuring features.[36] It also allows tracking in lighting conditions ranging from total darkness to very bright.
Another, less used, method is known as passive light. It uses visible light to illuminate, something which may cause some distractions to users.[35] Another challenge with this method is that the contrast of the pupil is less than in the active light methods, therefore, the center of iris is used for calculating the vector instead.[37] This calculation needs to detect the boundary of the iris and the white sclera (limbus tracking). It presents another challenge for vertical eye movements due to obstruction of eyelids.[38]
- Infrared / near-infrared: bright pupil.
- Infrared / near-infrared: dark pupil and corneal reflection.
- Visible light: center of iris (red), corneal reflection (green), and output vector (blue).
Eye-tracking setups vary greatly: some are head-mounted, some require the head to be stable (for example, with a chin rest), and some function remotely and automatically track the head during motion. Most use a sampling rate of at least 30 Hz. Although 50/60 Hz is more common, today many video-based eye trackers run at 240, 350 or even 1000/1250 Hz, speeds needed in order to capture fixational eye movements or correctly measure saccade dynamics.
Eye movements are typically divided into fixations and saccades – when the eye gaze pauses in a certain position, and when it moves to another position, respectively. The resulting series of fixations and saccades is called a scanpath. Smooth pursuit describes the eye following a moving object. Fixational eye movements include microsaccades: small, involuntary saccades that occur during attempted fixation. Most information from the eye is made available during a fixation or smooth pursuit, but not during a saccade.[39]
Scanpaths are useful for analyzing cognitive intent, interest, and salience. Other biological factors (some as simple as gender) may affect the scanpath as well. Eye tracking in human–computer interaction (HCI) typically investigates the scanpath for usability purposes, or as a method of input in gaze-contingent displays, also known as gaze-based interfaces.[40]
Data presentation
Interpretation of the data that is recorded by the various types of eye-trackers employs a variety of software that animates or visually represents it, so that the visual behavior of one or more users can be graphically resumed. The video is generally manually coded to identify the AOIs(Area Of Interests) or recently using artificial intelligence. Graphical presentation is rarely the basis of research results, since they are limited in terms of what can be analysed - research relying on eye-tracking, for example, usually requires quantitative measures of the eye movement events and their parameters, The following visualisations are the most commonly used:
Animated representations of a point on the interface This method is used when the visual behavior is examined individually indicating where the user focused their gaze in each moment, complemented with a small path that indicates the previous saccade movements, as seen in the image.
Static representations of the saccade path This is fairly similar to the one described above, with the difference that this is static method. A higher level of expertise than with the animated ones is required to interpret this.
Heat maps An alternative static representation, used mainly for the agglomerated analysis of the visual exploration patterns in a group of users. In these representations, the ‘hot’ zones or zones with higher density designate where the users focused their gaze (not their attention) with a higher frequency. Heat maps are the best known visualization technique for eyetracking studies.[41]
Blind zones maps, or focus maps This method is a simplified version of the Heat maps where the visually less attended zones by the users are displayed clearly, thus allowing for an easier understanding of the most relevant information, that is to say, we are informed about which zones were not seen by the users.
Saliency maps Similar to heat maps, a saliency map illustrates areas of focus by brightly displaying the attention-grabbing objects over an initially black canvas. The more focus is given to a particular object, the brighter it will appear.[42]
Eye-tracking vs. gaze-tracking
Eye-trackers necessarily measure the rotation of the eye with respect to some frame of reference. This is usually tied to the measuring system. Thus, if the measuring system is head-mounted, as with EOG or a video-based system mounted to a helmet, then eye-in-head angles are measured. To deduce the line of sight in world coordinates, the head must be kept in a constant position or its movements must be tracked as well. In these cases, head direction is added to eye-in-head direction to determine gaze direction.
If the measuring system is table-mounted, as with scleral search coils or table-mounted camera (“remote”) systems, then gaze angles are measured directly in world coordinates. Typically, in these situations head movements are prohibited. For example, the head position is fixed using a bite bar or a forehead support. Then a head-centered reference frame is identical to a world-centered reference frame. Or colloquially, the eye-in-head position directly determines the gaze direction.
Some results are available on human eye movements under natural conditions where head movements are allowed as well.[43] The relative position of eye and head, even with constant gaze direction, influences neuronal activity in higher visual areas.[44]
Practice
A great deal of research has gone into studies of the mechanisms and dynamics of eye rotation, but the goal of eye- tracking is most often to estimate gaze direction. Users may be interested in what features of an image draw the eye, for example. It is important to realize that the eye-tracker does not provide absolute gaze direction, but rather can measure only changes in gaze direction. In order to know precisely what a subject is looking at, some calibration procedure is required in which the subject looks at a point or series of points, while the eye tracker records the value that corresponds to each gaze position. (Even those techniques that track features of the retina cannot provide exact gaze direction because there is no specific anatomical feature that marks the exact point where the visual axis meets the retina, if indeed there is such a single, stable point.) An accurate and reliable calibration is essential for obtaining valid and repeatable eye movement data, and this can be a significant challenge for non-verbal subjects or those who have unstable gaze.
Each method of eye-tracking has advantages and disadvantages, and the choice of an eye-tracking system depends on considerations of cost and application. There are offline methods and online procedures like AttentionTracking. There is a trade-off between cost and sensitivity, with the most sensitive systems costing many tens of thousands of dollars and requiring considerable expertise to operate properly. Advances in computer and video technology have led to the development of relatively low-cost systems that are useful for many applications and fairly easy to use.[45] Interpretation of the results still requires some level of expertise, however, because a misaligned or poorly calibrated system can produce wildly erroneous data.
Eye-tracking while driving a car in a difficult situation
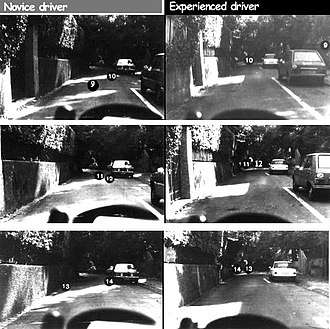
The eye movement of two groups of drivers have been filmed with a special head camera by a team of the Swiss Federal Institute of Technology: Novice and experienced drivers had their eye-movement recorded while approaching a bend of a narrow road. The series of images has been condensed from the original film frames[47] to show 2 eye fixations per image for better comprehension.
Each of these stills corresponds to approximately 0.5 seconds in realtime.
The series of images shows an example of eye fixations #9 to #14 of a typical novice and an experienced driver.
Comparison of the top images shows that the experienced driver checks the curve and even has Fixation No. 9 left to look aside while the novice driver needs to check the road and estimate his distance to the parked car.
In the middle images, the experienced driver is now fully concentrating on the location where an oncoming car could be seen. The novice driver concentrates his view on the parked car.
In the bottom image the novice is busy estimating the distance between the left wall and the parked car, while the experienced driver can use his peripheral vision for that and still concentrate his view on the dangerous point of the curve: If a car appears there, he has to give way, i. e. stop to the right instead of passing the parked car.[48]
Eye-tracking of younger and elderly people while walking
While walking, elderly subjects depend more on foveal vision than do younger subjects. Their walking speed is decreased by a limited visual field, probably caused by a deteriorated peripheral vision.
Younger subjects make use of both their central and peripheral vision while walking. Their peripheral vision allows faster control over the process of walking.[49]
Applications
A wide variety of disciplines use eye-tracking techniques, including cognitive science; psychology (notably psycholinguistics; the visual world paradigm); human-computer interaction (HCI); human factors and ergonomics; marketing research and medical research (neurological diagnosis). Specific applications include the tracking eye movement in language reading, music reading, human activity recognition, the perception of advertising, playing of sports, distraction detection and cognitive load estimation of drivers and pilots and as a means of operating computers by people with severe motor impairment.
Commercial applications
In recent years, the increased sophistication and accessibility of eye-tracking technologies have generated a great deal of interest in the commercial sector. Applications include web usability, advertising, sponsorship, package design and automotive engineering. In general, commercial eye-tracking studies function by presenting a target stimulus to a sample of consumers while an eye tracker is used to record the activity of the eye. Examples of target stimuli may include websites; television programs; sporting events; films and commercials; magazines and newspapers; packages; shelf displays; consumer systems (ATMs, checkout systems, kiosks); and software. The resulting data can be statistically analyzed and graphically rendered to provide evidence of specific visual patterns. By examining fixations, saccades, pupil dilation, blinks and a variety of other behaviors, researchers can determine a great deal about the effectiveness of a given medium or product. While some companies complete this type of research internally, there are many private companies that offer eye-tracking services and analysis.
One of the most prominent fields of commercial eye-tracking research is web usability. While traditional usability techniques are often quite powerful in providing information on clicking and scrolling patterns, eye-tracking offers the ability to analyze user interaction between the clicks and how much time a user spends between clicks, thereby providing valuable insight into which features are the most eye-catching, which features cause confusion and which are ignored altogether. Specifically, eye-tracking can be used to assess search efficiency, branding, online advertisements, navigation usability, overall design and many other site components. Analyses may target a prototype or competitor site in addition to the main client site.
Eye-tracking is commonly used in a variety of different advertising media. Commercials, print ads, online ads and sponsored programs are all conducive to analysis with current eye-tracking technology. For instance in newspapers, eye-tracking studies can be used to find out in what way advertisements should be mixed with the news in order to catch the reader's eyes. Analyses focus on visibility of a target product or logo in the context of a magazine, newspaper, website, or televised event. One example is an analysis of eye movements over advertisements in the Yellow Pages. The study focused on what particular features caused people to notice an ad, whether they viewed ads in a particular order and how viewing times varied. The study revealed that ad size, graphics, color, and copy all influence attention to advertisements. Knowing this allows researchers to assess in great detail how often a sample of consumers fixates on the target logo, product or ad. As a result, an advertiser can quantify the success of a given campaign in terms of actual visual attention.[50] Another example of this is a study that found that in a search engine results page, authorship snippets received more attention than the paid ads or even the first organic result.[51]
Safety applications
Scientists in 2017 constructed a Deep Integrated Neural Network (DINN) out of a Deep Neural Network and a convolutional neural network.[24] The goal was to use deep learning to examine images of drivers and determine their level of drowsiness by "classify[ing] eye states." With enough images, the proposed DINN could ideally determine when drivers blink, how often they blink, and for how long. From there, it could judge how tired a given driver appears to be, effectively conducting an eye-tracking exercise. The DINN was trained on data from over 2,400 subjects and correctly diagnosed their states 96%-99.5% of the time. Most other artificial intelligence models performed at rates above 90%.[24] This technology could ideally provide another avenue for driver drowsiness detection.
Game theory applications
In a 2019 study, a Convolutional Neural Network (CNN) was constructed with the ability to identify individual chess pieces the same way other CNNs can identify facial features.[26] It was then fed eye-tracking input data from thirty chess players of various skill levels. With this data, the CNN used gaze estimation to determine parts of the chess board to which a player was paying close attention. It then generated a saliency map to illustrate those parts of the board. Ultimately, the CNN would combine its knowledge of the board and pieces with its saliency map to predict the players' next move. Regardless of the training dataset the neural network system was trained upon, it predicted the next move more accurately than if it had selected any possible move at random, and the saliency maps drawn for any given player and situation were more than 54% similar.[26]
Assistive technology
People with severe motor impairment can use eye tracking for interacting with computers [52] as it is faster than single switch scanning techniques and intuitive to operate [53][54]. Motor impairment caused by Cerebral Palsy [55] or Amyotrophic lateral sclerosis often affects speech, and users with Severe Speech and Motor Impairment (SSMI) use a type of software known as Augmentative and Alternative Communication (AAC) aid [56], that displays icons, words and letters on screen [57] and uses text-to-speech software to generate spoken output [58]. In recent times, researchers also explored eye tracking to control robotic arm [59] and powered wheelchair [60]. Eye tracking is also helpful in analysing visual search patterns [61], detecting presence of Nystagmus and detecting early signs of learning disability by analysing eye gaze movement during reading [62].
Aviation applications
Eye tracking was already studied for flight safety by comparing scan paths and fixation duration to evaluate the progress of pilot trainees [63] , for estimating pilots’ skills [64] , for analyzing crew’s joint attention and shared situational awareness. Eye tracking technology was also explored to interact with helmet mounted display systems [65] and multi-functional displays [66] in military aircraft. Studies were conducted to investigate the utility of eye tracker for Head-up target locking and Head-up target acquisition in Helmet mounted display systems (HMDS). Pilots' feedback suggested that even though the technology is promising, its hardware and software components are yet to be matured. Research on interacting with multi-functional displays in simulator environment showed that eye tracking can improve the response times and perceived cognitive load significantly over existing systems. Further, research also investigated utilizing measurements of fixation and pupillary responses to estimate pilot's cognitive load. Estimating cognitive load can help to design next generation adaptive cockpits with improved flight safety [67] .
Automotive applications
In recent time, eye tracking technology is investigated in automotive domain in both passive and active ways. National Highway Traffic Safety Administration measured glance duration for undertaking secondary tasks while driving and used it to promote safety by discouraging the introduction of excessively distracting devices in vehicles. [68]. In addition to distraction detection, eye tracking is also used to interact with IVIS [69]. Though initial research [70] investigated the efficacy of eye tracking system for interaction with HDD (Head Down Display), it still required drivers to take their eyes off the road while performing a secondary task. Recent studies investigated eye gaze controlled interaction with HUD (Head Up Display) that eliminates eyes-off-road distraction [71]. Eye tracking is also used to monitor cognitive load of drivers to detect potential distraction. Though researchers [72] explored different methods to estimate cognitive load of drivers from different physiological parameters, usage of ocular parameters explored a new way to use the existing eye trackers to monitor cognitive load of drivers in addition to interaction with IVIS [73] , [74].
The future of eye tracking and user experience[75]
It might be difficult for those in the field of user experience (UX) design to imagine what we will be doing in the next decade. Many of us have become comfortable as UX practitioners with our existing methodologies and have been able to refine our processes over the last two decades. New game-changing capabilities are on their way that may redefine how we conduct our research.
Integrating eye tracking into user-centered design methodology
In the past, user research was considered a luxury and an optional component of digital product design. Over time, product managers found ways to successfully integrate user-centered design into most projects. To do this, the process needed to be more agile and compatible with often hectic development cycles.
Eye tracking has always been seen as the ultimate in luxury in both time and money. Few user research labs had eye trackers, and many of those who did had few opportunities to use them. The management and design teams also tended to see limited value from eye-tracking studies; the perceived benefit was often that they would receive pretty pictures with little interpretation beyond where users did or did not look.
While still in its infancy, eye tracking has made its way into user research in recent years.
We are slowly seeing the emergence of eye-tracking research that can scale to quick turnaround sutides with smaller sample sizes to larger scale studies that provide deeper insights into user behavior. Education continues with product design teams regarding limitations and opportunities for small-scale qualitative eye-tracking studies. These studies have emerged as a compromise of sorts that allow eye tracking to fit into tight development time lines but can be highly focused to certain interface components rather than a holistic view of the entire product experience.
Larger eye-tracking studies are now supported, mostly by larger corporations, to help understand users as part of a comprehensive metrics program. Companies are beginning to understand the value in using eye-tracking insights as a part of a continual improvement process where small, iterative changes can be made to improve the user experience.
Continued Improvements to Eye-Tracking Technology
Current uses of eye tracking are often limited to a lab environment where we set up an artificial situation for study participants. Yet still, eye tracking has come a long way and is now easy to use and not very intrusive. We can now conduct studies in which participants are hardly aware of the eye tracker at all. We suspect that the technology will continue to improve and will be less and less of an obstacle to collect eye-tracking data.
New eye trackers, such as the Tobii X2, allow for true plug and play capability. These near pocket-sized models can be connected to most computers with a single connector, such as a USB cable. These "mini" eye trackers are also incredibly versatile in the possible types of testing configurations including laptops, desktop displays, movile devices, and even print materials.
The usability of eye-trackings software has improved almost as well as the hardware. The current version of most eye-tracking software is considerably easier to use and is more stable, with fewer crashes. There has also been a concerted effort to make the software hardware agnostic allowing researchers to mix and match configurations that best meet their study needs. Eye-tracking manufacturers have vastly improved the ability to track individuals with different physical attributes in a variety of environmental conditions. This has helped to drastically reduce the number of individuals that cannot be tracked and has reduced the need to over-recruit participants.
The current trajectory of software and hardware improvements will likely lead to near effortless abilities to collect eye-tracking data by 2020. This will make eye tracking accessible to a wider range of professionals and will help improve the turnaround time of results.
While software and hardware continue to improve, UX researchers must also improve their study techniques and interpretation of eye-tracking findings. This is arguably more important than the improvement in technology; a poorly conducted study and misused eye-tracking data can only harm the field. In the future, advances in artificial intelligence will help by leading to low-level results that are analyzed and processed by computers, allowing UX researcher to focus less on interpreting individual fixation patterns and descriptive statistics and more on strategic implications.
The future of the Eye-Tracking Technology
Physiological response measurements coupled with eye tracking have entered the UX field. These techniques are in their infancy for UX researchers, but we expect these technologies to continue to improve and be as easy to use as a modern eye trackers. By 2020, companies large and small will be more aggressive in seeking new ways to engage their users, and it will be common for marketers and UX practitioners to use eye tracking with electroencephalography or electrodermal activity to assess the user experience. Competition in the digital advertising space will go beyond traditional website banner ads and click-through rates. While much of the rich user experience still involves traditional links, innovative products are emerging that allow UX researchers new opportunities.
Current eye-tracking technology allows UX researcher to track users' eyes through untethered headset units, such as the Tobii Glasses. These eye trackers allow users complete freedom of movement and can track everything the user looks at from mobile devices to the menu in their local café. By allowing users to freely move about their environment, we are able to observe more natural behaviors compared to when participants sit in front of an eye tracker in a lab. Marketeres are now able to overlay eye-tracking data with shopping shelves to assess what people look at while they shop, and Google has recently patented a process for measuring pay-per-gaze as a way to measure the effectiveness of advertising. The patent describes a process in which a head-mounted gaze-tracking device can identify ads that a user looks. These data can be used to understand what types of advertisements people attend to and in what situations people pay attention to them. Advertisements will likely continue to be a revenue source for both digital and offline content in the future. Eye tracking will help designers to understand a user's level of engagement with ads by fixation counts and duration in the context of the user's actions.
Others are creating technology whereby the eyes control the device. Taking advantage of built-in webcam technology, manufactureres are introducing eye-tracking capabilities into mobile devices. A Wall Street Journal article published in May 2013 broke the news that Amazon has been developing a new mobile phone that would include retina-tracking technology that will allow users to navigate through content by simply using their eyes. Software already exists that allows users to merely look at the corner of an iPad to turn the page of an eBook, or look at the corner of a television screen to change the station. This modern technology enables accessibility for disabled people who cannot interact with technology with their hands. The smartwatch has recently been patented, which uses eye trackers to decide what content to display on a tiny watch interface, and other researchers are using eye tracking to diagnose Alzheimer's disease, schizophrenia, and memory disorders. Thus, we are now at a time when eye tracking is used not only to evaluate products but to interact with them and to diagnose disorders.
As eye-tracking technology continues to become miniaturized and cost less, we will see many more devices with this capability built in. Google Glass presents numerous opportunities for interaction designers ans user researchers. While the first generation of this technology will be somewhat limited, by 2020, we can expect these types of devices to be commonplace. Eye tracking is likely to become an integral part of head-mounted displays where most user interactions will take place with eye movements. In addition to being an input device, user researchers can see through the eyes of their users with the existing external camera and track where they are looking with a camera facing the eye.
Ubiquitous Eye Tracking
New technologies are emerging that will allow UX researchers to track users' eyes anywhere and everywhere. Obviously this will come with significant concerns about privacy and the ability for the user to control when they are being tracked.
While webcam-based eye tracking has emerged, there are still significant limitations to using this technology. Aga Bojko[76] (2013) identified several concerns about this methodology related to accuracy:
"Webcam eye tracking has much lower accuracy than real eye trackers. While a typical remote eye tracker (Tobii T60) has accuracy of 0.5 degrees of visual angle, a webcam will produce accuracy of 2-5 degrees, provided that the participant is NOT MOVING. To give you an idea of what that means, five degrees correspond to 2.5 inches (6 cm) on a computer monitor (assuming viewing distance of 27 inches), so the actual gaze location could be anywhere within a radius of 2.5 inches from the gaze location recorded with a webcam. I don't know about you but I wouldn't be comfortable with that level of inaccuracy in my studies".
As both the quality of webcams increases and the algorithms used for tracking the movement of the eyes improves, we can expect to see more of this type of eye tracking used in the future.
A short distance in the future will be a time of ubiquitous eye tracking with the ability to track a user's eyes on nearly every device they use. For UX researchers, this opens up enormous opportunities for large-scale quantitative eye-tracking studies. In addition to reaching broad audience groups currently not practical with today's technology, we will also have true freedom to collect eye-tracking data in the user's natural environment.
Taking the next step
Currently eye-tracking is used by industry professionals to assess and improve the user experience of websites, forms, surveys, and games, both on desktop and mobile devices. The case studies and topics the wide range of applications of eye tracking in user experience design. It's by no means an exhaustive assembly of all possible uses for eye tracking and interactive media. Many of the applications referenced are still their infancy and further research is required to better understand these areas.
See also
- AttentionTracking
- Eye movement
- Eye movement in language reading
- Eye movement in music reading
- Eye tracking on the ISS
- Fovea
- Foveated imaging
- Gaze-contingency paradigm
- Marketing research
- Mouse-Tracking
- Peripheral vision
- Saccade
- Screen reading
- visage SDK
Notes
- Reported in Huey 1908/1968.
- Huey, Edmund. The Psychology and Pedagogy of Reading (Reprint). MIT Press 1968 (originally published 1908).
- Buswell (1922, 1937)
- (1935)
- Yarbus 1967
- Yarbus 1967, p. 190
- Yarbus 1967, p. 194
- Yarbus 1967, p. 191
- Yarbus 1967, p. 193
- Hunziker, H. W. (1970). Visuelle Informationsaufnahme und Intelligenz: Eine Untersuchung über die Augenfixationen beim Problemlösen. Schweizerische Zeitschrift für Psychologie und ihre Anwendungen, 1970, 29, Nr 1/2 (english abstract: http://www.learning-systems.ch/multimedia/forsch1e.htm )
- http://www.learning-systems.ch/multimedia/eye%5B%5D movements problem solving.swf
- "Visual Perception: Eye Movements in Problem Solving". www.learning-systems.ch. Retrieved 9 October 2018.
- Rayner (1978)
- Just and Carpenter (1980)
- Posner (1980)
- Wright & Ward (2008)
- Robert J. K. Jacob; Keith S. Karn (2003). "Eye Tracking in Human–Computer Interaction and Usability Research: Ready to Deliver the Promises". In Hyona; Radach; Deubel (eds.). The Mind's Eye: Cognitive and Applied Aspects of Eye Movement Research. Oxford, England: Elsevier Science BV. CiteSeerX 10.1.1.100.445. ISBN 0-444-51020-6.
- Schiessl, Michael; Duda, Sabrina; Thölke, Andreas; Fischer, Rico. "Eye tracking and its application in usability and media research" (PDF).
- Hoffman 1998
- Deubel, Heiner (1996). "Saccade target selection and object recognition: Evidence for a common attentional mechanism". Vision Research. 36 (12): 1827–1837. doi:10.1016/0042-6989(95)00294-4. PMID 8759451.
- Holsanova 2007
- Cognolato M, Atzori M, Müller H (2018). "Head-mounted eye gaze tracking devices: An overview of modern devices and recent advances". Journal of Rehabilitation and Assistive Technologies Engineering. 5: 205566831877399. doi:10.1177/2055668318773991. PMC 6453044. PMID 31191938.
- Alexander, Robert; Macknik, Stephen; Martinez-Conde, Susana (2020). "Microsaccades in applied environments: Real-world applications of fixational eye movement measurements". Journal of Eye Movement Research. 12 (6). doi:10.16910/jemr.12.6.15.
- Zhao, Lei; Wang, Zengcai; Zhang, Guoxin; Qi, Yazhou; Wang, Xiaojin (15 November 2017). "Eye state recognition based on deep integrated neural network and transfer learning". Multimedia Tools and Applications. 77 (15): 19415–19438. doi:10.1007/s11042-017-5380-8. ISSN 1380-7501.
- Stember, J. N.; Celik, H.; Krupinski, E.; Chang, P. D.; Mutasa, S.; Wood, B. J.; Lignelli, A.; Moonis, G.; Schwartz, L. H.; Jambawalikar, S.; Bagci, U. (August 2019). "Eye Tracking for Deep Learning Segmentation Using Convolutional Neural Networks". Journal of Digital Imaging. 32 (4): 597–604. doi:10.1007/s10278-019-00220-4. ISSN 0897-1889. PMC 6646645. PMID 31044392.
- Louedec, Justin Le; Guntz, Thomas; Crowley, James L.; Vaufreydaz, Dominique (2019). "Deep learning investigation for chess player attention prediction using eye-tracking and game data". Proceedings of the 11th ACM Symposium on Eye Tracking Research & Applications - ETRA '19. New York, New York, USA: ACM Press: 1–9. arXiv:1904.08155. Bibcode:2019arXiv190408155L. doi:10.1145/3314111.3319827. ISBN 978-1-4503-6709-7.
- Lian, Dongze; Hu, Lina; Luo, Weixin; Xu, Yanyu; Duan, Lixin; Yu, Jingyi; Gao, Shenghua (October 2019). "Multiview Multitask Gaze Estimation With Deep Convolutional Neural Networks". IEEE Transactions on Neural Networks and Learning Systems. 30 (10): 3010–3023. doi:10.1109/TNNLS.2018.2865525. ISSN 2162-237X. PMID 30183647.
- David A. Robinson: A method of measuring eye movement using a scleral search coil in a magnetic field, IEEE Transactions on Bio-Medical Electronics, October 1963, 137–145 (PDF)
- Crane, H.D.; Steele, C.M. (1985). "Generation-V dual-Purkinje-image eyetracker". Applied Optics. 24 (4): 527–537. Bibcode:1985ApOpt..24..527C. doi:10.1364/AO.24.000527. PMID 18216982. S2CID 10595433.
- Elbert, T., Lutzenberger, W., Rockstroh, B., Birbaumer, N., 1985. Removal of ocular artifacts from the EEG. A biophysical approach to the EOG. Electroencephalogr Clin Neurophysiol 60, 455-463.
- Keren, A.S.; Yuval-Greenberg, S.; Deouell, L.Y. (2010). "Saccadic spike potentials in gamma-band EEG: Characterization, detection and suppression". NeuroImage. 49 (3): 2248–2263. doi:10.1016/j.neuroimage.2009.10.057. PMID 19874901.
- Bulling, A.; Roggen, D.; Tröster, G. (2009). "Wearable EOG goggles: Seamless sensing and context-awareness in everyday environments". Journal of Ambient Intelligence and Smart Environments. 1 (2): 157–171. doi:10.3233/AIS-2009-0020. hdl:20.500.11850/352886.
- Sopic, D., Aminifar, A., & Atienza, D. (2018). e-glass: A wearable system for real-time detection of epileptic seizures. In IEEE International Symposium on Circuits and Systems (ISCAS).
- Witzner Hansen, Dan; Qiang Ji (March 2010). "In the Eye of the Beholder: A Survey of Models for Eyes and Gaze". IEEE Trans. Pattern Anal. Mach. Intell. 32 (3): 478–500. doi:10.1109/tpami.2009.30. PMID 20075473.
- Gneo, Massimo; Schmid, Maurizio; Conforto, Silvia; D’Alessio, Tommaso (2012). "A free geometry model-independent neural eye-gaze tracking system". Journal of NeuroEngineering and Rehabilitation. 9 (1): 82. doi:10.1186/1743-0003-9-82. PMC 3543256. PMID 23158726.
- The Eye: A Survey of Human Vision; Wikimedia Foundation
- Sigut, J; Sidha, SA (February 2011). "Iris center corneal reflection method for gaze tracking using visible light". IEEE Transactions on Bio-Medical Engineering. 58 (2): 411–9. doi:10.1109/tbme.2010.2087330. PMID 20952326.
- Hua, H; Krishnaswamy, P; Rolland, JP (15 May 2006). "Video-based eyetracking methods and algorithms in head-mounted displays". Optics Express. 14 (10): 4328–50. Bibcode:2006OExpr..14.4328H. doi:10.1364/oe.14.004328. PMID 19516585.
- Purves, D; st al. (2001). Neuroscience, 2d ed. Sunderland (MA): Sinauer Assocs. p. What Eye Movements Accomplish.
- Majaranta, P., Aoki, H., Donegan, M., Hansen, D.W., Hansen, J.P., Hyrskykari, A., Räihä, K.J., Gaze Interaction and Applications of Eye Tracking: Advances in Assistive Technologies, IGI Global, 2011
- Nielsen, Jakob. Pernice, Kara. (2010). " Eyetracking Web Usability." New Rideres Publishing. p. 11. ISBN 0-321-49836-4. Google Book Search. Retrieved on 28 October 2013.
- Le Meur, O; Baccino, T (2013). "Methods for comparing scanpaths and saliency maps: strengths and weaknesses". Behavior Research Methods. 45 (1).
- Einhäuser, W; Schumann, F; Bardins, S; Bartl, K; Böning, G; Schneider, E; König, P (2007). "Human eye-head co-ordination in natural exploration". Network: Computation in Neural Systems. 18 (3): 267–297. doi:10.1080/09548980701671094. PMID 17926195.
- Andersen, R. A.; Bracewell, R. M.; Barash, S.; Gnadt, J. W.; Fogassi, L. (1990). "Eye position effects on visual, memory, and saccade-related activity in areas LIP and 7a of macaque" (PDF). Journal of Neuroscience. 10 (4): 1176–1196. doi:10.1523/JNEUROSCI.10-04-01176.1990.
- Ferhat, Onur; Vilariño, Fernando (2016). "Low Cost Eye Tracking: The Current Panorama". Computational Intelligence and Neuroscience. 2016: 1–14. doi:10.1155/2016/8680541. PMC 4808529. PMID 27034653.
- Hans-Werner Hunziker, (2006) Im Auge des Lesers: foveale und periphere Wahrnehmung - vom Buchstabieren zur Lesefreude [In the eye of the reader: foveal and peripheral perception - from letter recognition to the joy of reading] Transmedia Stäubli Verlag Zürich 2006 ISBN 978-3-7266-0068-6 Based on data from:Cohen, A. S. (1983). Informationsaufnahme beim Befahren von Kurven, Psychologie für die Praxis 2/83, Bulletin der Schweizerischen Stiftung für Angewandte Psychologie
- Cohen, A. S. (1983). Informationsaufnahme beim Befahren von Kurven, Psychologie für die Praxis 2/83, Bulletin der Schweizerischen Stiftung für Angewandte Psychologie
- Pictures from: Hans-Werner Hunziker, (2006) Im Auge des Lesers: foveale und periphere Wahrnehmung – vom Buchstabieren zur Lesefreude [In the eye of the reader: foveal and peripheral perception – from letter recognition to the joy of reading] Transmedia Stäubli Verlag Zürich 2006 ISBN 978-3-7266-0068-6
- Itoh, Nana; Fukuda, Tadahiko (2002). "Comparative Study of Eye Movements in Extent of Central and Peripheral Vision and Use by Young and Elderly Walkers". Perceptual and Motor Skills. 94 (3_suppl): 1283–1291. doi:10.2466/pms.2002.94.3c.1283. PMID 12186250.
- Lohse, Gerald; Wu, D. J. (1 February 2001). "Eye Movement Patterns on Chinese Yellow Pages Advertising". Electronic Markets. 11 (2): 87–96. doi:10.1080/101967801300197007. S2CID 1064385.CS1 maint: ref=harv (link)
- "Eye Tracking Study: The Importance of Using Google Authorship in Search Results"
- {cite journal|last1 = Corno |first1= F. |last2= Farinetti |first2= L.|last3= Signorile |first3= I.|date= August 2002|title=A cost-effective solution for eye-gaze assistive technology|url= https://ieeexplore.ieee.org/abstract/document/1035632%7Cjournal= IEEE International Conference on Multimedia and Expo |vol.= 2|pages= 433-436 |access-date= 5 August 2020}}
- Pinheiro, C.; Naves, E. L.; Pino, P.; Lesson, E.; Andrade, A.O.; Bourhis, G. (July 2011). "Alternative communication systems for people with severe motor disabilities: a survey". Biomedical engineering online. 10 (1): 1–28. Retrieved 5 August 2020.
- Saunders, M.D.; Smagner, J.P.; Saunders, R.R. (August 2003). "Improving methodological and technological analyses of adaptive switch use of individuals with profound multiple impairments". Behavioral Interventions: Theory & Practice in Residential & Community‐Based Clinical Programs. 18 (4): 227–243. Retrieved 5 August 2020.
- "Cerebral Palsy (CP)". Retrieved 4 August 2020.
- Wilkinson, K.M.; Mitchell, T. (March 2014). "Eye tracking research to answer questions about augmentative and alternative communication assessment and intervention". Augmentative and Alternative Communication. 30 (2): 106–119. Retrieved 5 August 2020.
- Galante, A.; Menezes, P. (June 2012). "A gaze-based interaction system for people with cerebral palsy". Procedia Technology. 5: 895–902. Retrieved 3 August 2020.
- BLISCHAK, D.; LOMBARDINO, L.; DYSON, A. (June 2003). "Use of speech-generating devices: In support of natural speech". Augmentative and alternative communication. 19 (1): 29–35. Retrieved 5 August 2020.
- Sharma, V.K.; Murthy, L. R. D.; Singh Saluja, K.; Mollyn, V.; Sharma, G.; Biswas, Pradipta (August 2020). "Webcam controlled robotic arm for persons with SSMI". Technology and Disability. 32 (3): 1–19. Retrieved 5 August 2020.
- Eid, M.A.; Giakoumidis, N.; El Saddik, A. (July 2016). "A novel eye-gaze-controlled wheelchair system for navigating unknown environments: case study with a person with ALS". IEEE Access. 4: 558–573. Retrieved 4 August 2020.
- Jeevithashree, D. V.; Saluja, K.S.; Biswas, Pradipta (December 2019). "A case study of developing gaze-controlled interface for users with severe speech and motor impairment". Technology and Disability. 31(1-2): 63–76. Retrieved 5 August 2020.
- Jones, M.W.; Obregón, M.; Kelly, M.L.; Branigan, H.P. (May 2008). "Elucidating the component processes involved in dyslexic and non-dyslexic reading fluency: An eye-tracking study". Cognition. 109 (3): 389–407. Retrieved 5 August 2020.
- Calhoun, G. L; Janson (1991). "Eye line-of-sight control compared to manual selection of discrete switches". Armstrong Laboratory Report AL-TR-1991-0015.
|access-date=
requires|url=
(help) - Fitts, P.M.; Jones, R.E.; Milton, J.L (1950). "Eye movements of aircraft pilots during instrument-landing approaches". Aeronaut. Eng. Rev. Retrieved 20 July 2020.
- De Reus, A. J. C.; Zon, R.; Ouwerkerk, R. (2012). "Exploring the use of an eye tracker in a helmet mounted display". Retrieved 31 July 2020. Cite journal requires
|journal=
(help) - DV, JeevithaShree; Murthy,, L R.D.; Saluja, K. S.; Biswas, P. (2018). "Operating different displays in military fast jets using eye gaze tracker". Journal of Aviation Technology and Engineering. 8 (4). Retrieved 24 July 2020.CS1 maint: extra punctuation (link)
- Babu, M.; D V, JeevithaShree; Prabhakar, G.; Saluja, K.P.; Pashilkar, A.; Biswas, P. (2019). "Estimating pilots' cognitive load from ocular parameters through simulation and in-flight studies". journal of Eye Movement Research. 12 (3). Retrieved 3 August 2020.
- "Visual-Manual NHTSA Driver Distraction Guidelines for In-Vehicle Electronic Devices".
- Mondragon, C. K.; Bleacher, B. (2013). "Eye tracking control of vehicle entertainment systems". Retrieved 3 August 2020. Cite journal requires
|journal=
(help) - Poitschke, T.; Laquai, F.; Stamboliev, S.; Rigoll, G. (2011). "Gaze-based interaction on multiple displays in an automotive environment". IEEE International Conference on Systems, Man, and Cybernetics (SMC): 543–548. ISSN 1062-922X. Retrieved 3 August 2020.
- Prabhakar, G.; Ramakrishnan, A.; Murthy, L.; Sharma, V.K.; Madan, M.; Deshmukh, S.; Biswas, P. (2019). "Interactive Gaze & Finger controlled HUD for Cars". Journal of Multimodal User Interface. Retrieved 3 August 2020.
- Marshall, S. (2002). "The index of cognitive activity: Measuring cognitive workload". In Proc. 7th conference on human factors and power plants. Retrieved 3 August 2020.
- Duchowski, A. T.; Biele, C.; Niedzielska, A.; Krejtz, K.; Krejtz, I.; Kiefer, P.; Raubal, M.; Giannopoulos, I. (2018). "The Index of Pupillary Activity Measuring Cognitive Load vis-à-vis Task Difficulty with Pupil Oscillation". ACM SIGCHI Conference on Human Factors. Retrieved 3 August 2020.
- Prabhakar, G.; Mukhopadhyay, A.; MURTHY, L.; Modiksha, M. A. D. A. N.; Biswas, P. (2020). "Cognitive load estimation using Ocular Parameters in Automotive". Transportation Engineering. Retrieved 3 August 2020.
- Romano Bergstrom, Jennifer (2014). Eye Tracking in User Experience Design. Morgan Kaufmann. ISBN 978-0-12-408138-3.
- Bojko, Aga (2013). Eye Tracking The User Experience (A Practical Guide to Research). Rosenfeld Media. ISBN 978-1-933820-10-1.
References
![]() |
Wikimedia Commons has media related to Eye tracking. |
- Cornsweet, TN; Crane, HD (1973). "Accurate two-dimensional eye tracker using first and fourth Purkinje images". J Opt Soc Am. 63 (8): 921–8. Bibcode:1973JOSA...63..921C. doi:10.1364/josa.63.000921. PMID 4722578.
- Cornsweet, TN (1958). "New technique for the measurement of small eye movements". JOSA. 48 (11): 808–811. Bibcode:1958JOSA...48..808C. doi:10.1364/josa.48.000808. PMID 13588456.
- Just, MA; Carpenter, PA (1980). "A theory of reading: from eye fixation to comprehension" (PDF). Psychol Rev. 87 (4): 329–354. doi:10.1037/0033-295x.87.4.329.
- Rayner, K (1978). "Eye movements in reading and information processing". Psychological Bulletin. 85 (3): 618–660. CiteSeerX 10.1.1.294.4262. doi:10.1037/0033-2909.85.3.618. PMID 353867.
- Rayner, K (1998). "Eye movements in reading and information processing: 20 years of research". Psychological Bulletin. 124 (3): 372–422. CiteSeerX 10.1.1.211.3546. doi:10.1037/0033-2909.124.3.372. PMID 9849112.
- Romano Bergstrom, Jennifer (2014). Eye Tracking in User Experience Design. Morgan Kaufmann. ISBN 978-0-12-408138-3.
- Bojko, Aga (2013). Eye Tracking The User Experience (A Practical Guide to Research). Rosenfeld Media. ISBN 978-1-933820-10-1.
Commercial eye tracking
- Pieters, R.; Wedel, M. (2007). "Goal Control of Visual Attention to Advertising: The Yarbus Implication". Journal of Consumer Research. 34 (2): 224–233. CiteSeerX 10.1.1.524.9550. doi:10.1086/519150.
- Pieters, R.; Wedel, M. (2004). "Attention Capture and Transfer by elements of Advertisements". Journal of Marketing. 68 (2): 36–50. CiteSeerX 10.1.1.115.3006. doi:10.1509/jmkg.68.2.36.27794.