Serial time-encoded amplified microscopy
Serial time-encoded amplified imaging/microscopy or stretched time-encoded amplified imaging/microscopy' (STEAM) is a fast real-time optical imaging method that provides MHz frame rate, ~100 ps shutter speed, and ~30 dB (× 1000) optical image gain. Based on the Photonic Time Stretch technique, STEAM holds world records for shutter speed and frame rate in continuous real-time imaging. STEAM employs the Photonic Time Stretch with internal Raman amplification to realize optical image amplification to circumvent the fundamental trade-off between sensitivity and speed that affects virtually all optical imaging and sensing systems. This method uses a single-pixel photodetector, eliminating the need for the detector array and readout time limitations. Avoiding this problem and featuring the optical image amplification for dramatic improvement in sensitivity at high image acquisition rates, STEAM's shutter speed is at least 1000 times faster than the state-of-the-art CCD[1] and CMOS[2] cameras. Its frame rate is 1000 times faster than fastest CCD cameras and 10-100 times faster than fastest CMOS cameras.
History
The system combined photonic time stretch with internal Raman amplification, a technology developed earlier to create a femtosecond real-time single-shot digitizer[3] and a single shot stimulated Raman spectrometer [4] with the concept of spectral encoding. The first demonstration was a one-dimensional version[5] and later a two-dimensional version.[6] Recently, a fast imaging vibrometer was created by extending the system to an interferometric configuration.[7] The technology was then extended to time stretch quantitive phase imaging (TS-QPI) for label free classification of blood cells and combined with artificial intelligence (AI) for classification of cancer cells in blood with over 96% accuracy.[8] The system measured 16 biophysical parameters of cells simultaneously in single shot and performed hyper-dimensional classification using a Deep Neural Network (DNN). The results were compared with other machine learning classification algorithms such as logistic regression and naive Bayes with highest accuracy obtained with deep learning.
Background
Fast real-time optical imaging technology is indispensable for studying dynamical events such as shockwaves, laser fusion, chemical dynamics in living cells, neural activity, laser surgery, microfluidics, and MEMS. The usual techniques of conventional CCD and CMOS cameras are inadequate for capturing fast dynamical processes with high sensitivity and speed; there are technological limitations—it takes time to read out the data from the sensor array and there's a fundamental trade-off between sensitivity and speed: at high frame rates, fewer photons are collected during each frame, a problem that affects nearly all optical imaging systems.
The streak camera, used for diagnostics in laser fusion, plasma radiation, and combustion, operates in burst mode only (providing just several frames) and requires synchronization of the camera with the event to be captured. It is therefore unable to capture random or transient events in biological systems. Stroboscopes have a complementary role: they can capture the dynamics of fast events—but only if the event is repetitive, such as rotations, vibrations, and oscillations. They are unable to capture non-repetitive random events that occur only once or do not occur at regular intervals.
Principle of operation
The basic principle involve two steps both performed optically. In the first step, the spectrum of a broadband optical pulse is converted by a spatial disperser into a rainbow that illuminates the target. Here the rainbow pulse consists of many subpulses of different colors (frequencies), indicating that the different frequency components (colors) of the rainbow pulse are incident onto different spatial coordinates on the object. Therefore, the spatial information (image) of the object is encoded into the spectrum of the resultant reflected or transmitted rainbow pulse. The image-encoded reflected or transmitted rainbow pulse returns to the same spatial disperser or enters another spatial disperser to combine the colors of the rainbow back into a single pulse. Here STEAM's shutter speed or exposure time corresponds to the temporal width of the rainbow pulse. In the second step, the spectrum is mapped into a serial temporal signal that is stretched in time using dispersive Fourier transform to slow it down such that it can be digitized in real-time. The time stretch happens inside a dispersive fiber that is pumped to create internal Raman amplification. Here the image is optically amplified by stimulated Raman scattering to overcome the thermal noise level of the detector. The amplified time stretched serial image stream is detected by a single-pixel photodetector and the image is reconstructed in the digital domain. Subsequent pulses capture repetitive frames hence the laser pulse repetition rate corresponds to the frame rate of STEAM. The second is known as the time stretch analog-to-digital converter, otherwise known as the time stretch recording scope (TiSER).
Amplified dispersive Fourier transformation
The simultaneous stretching and amplification is also known as amplified time stretch dispersive Fourier transformation (TS-DFT).[9][10] The amplified time stretch technology was developed earlier to demonstrate analog-to-digital conversion with femtosecond real-time sampling rate [3] and to demonstrate stimulated Raman spectroscopy in single shot at millions of frames per second.[4] Amplified time stretch is a process in which the spectrum of an optical pulse is mapped by large group-velocity dispersion into a slowed down temporal waveform and amplified simultaneously by the process of stimulated Raman scattering. Consequently, the optical spectrum can be captured with a single-pixel photodetector and digitized in real-time. Pulses are repeated for repetitive measurements of the optical spectrum. Amplified time stretch DFT consists of a dispersive fiber pumped by lasers and wavelength-division multiplexers that couple the lasers into and out of the dispersive fiber. Amplified dispersive Fourier transformation was originally developed to enable ultra wideband analog to digital converters and has also been used for high throughput real-time spectroscopy. The resolution of STEAM imager is mainly determined by diffraction limit, sampling rate of the back-end digitizer, and spatial dispersers.[11]
Time stretch quantitative phase imaging
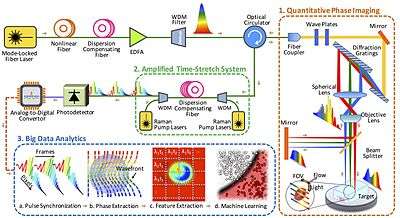
Time-stretch quantitative phase imaging (TS-QPI) is an imaging technique based on time-stretch technology for simultaneous measurement of phase and intensity spatial profiles.[12][13][14][15] Developed at UCLA, it has led to the development of time stretch artificial intelligence microscope.[12]
Time stretched imaging
In time stretched imaging, the object’s spatial information is encoded in the spectrum of laser pulses within a pulse duration of sub-nanoseconds. Each pulse representing one frame of the camera is then stretched in time so that it can be digitized in real-time by an electronic analog-to-digital converter (ADC). The ultra-fast pulse illumination freezes the motion of high-speed cells or particles in flow to achieve blur-free imaging. Detection sensitivity is challenged by the low number of photons collected during the ultra-short shutter time (optical pulse width) and the drop in the peak optical power resulting from the time stretch.[16] These issues are solved in time stretch imaging by implementing a low noise-figure Raman amplifier within the dispersive device that performs time stretching. Moreover, warped stretch transform can be used in time stretch imaging to achieve optical image compression and nonuniform spatial resolution over the field-of-view.
In the coherent version of the time-stretch camera, the imaging is combined with spectral interferometry to measure quantitative phase[17][18] and intensity images in real-time and at high throughput. Integrated with a microfluidic channel, coherent time stretch imaging system measures both quantitative optical phase shift and loss of individual cells as a high-speed imaging flow cytometer, capturing millions of line-images per second in flow rates as high as a few meters per second, reaching up to hundred-thousand cells per second throughput. The time stretch quantitative phase imaging can be combined with machine learning to achieve very accurate label-free classification of the cells.
Applications
This method is useful for a broad range of scientific, industrial, and biomedical applications that require high shutter speeds and frame rates. The one-dimensional version can be employed for displacement sensing, barcode reading, and blood screening;[19] the two-dimensional version for real-time observation, diagnosis, and evaluation of shockwaves, microfluidic flow,[20] neural activity, MEMS,[21] and laser ablation dynamics. The three-dimensional version is useful for range detection, dimensional metrology, and surface vibrometry and velocimetry.[22]
Image compression in optical domain
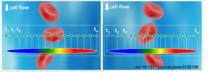
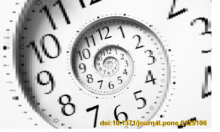
Big data not only brings opportunity, but also a challenge in biomedical and scientific instruments, whose acquisition and processing units are overwhelmed by a torrent of data. The need to compress massive volumes of data in real-time has fueled interest in nonuniform stretch transformations – operations that reshape the data according to its sparsity.
Recently researchers at UCLA demonstrated image compression performed in the optical domain and in real-time.[23] Using nonlinear group delay dispersion and time-stretch imaging, they were able to optically warp the image such that the information-rich portions are sampled at a higher sample density than the sparse regions. This was done by restructuring the image before optical-to-electrical conversion followed by a uniform electronic sampler. The reconstruction of the nonuniformly stretched image demonstrates that the resolution is higher where information is rich and lower where information is much less and relatively not important. The information-rich region at the center is well preserved while maintaining the same sampling rates compared to uniform case without down-sampling. Image compression was demonstrated at 36 million frames per second in real-time.
See also
- Charge-coupled device
- Time-resolved spectroscopy
- Time stretch dispersive Fourier transform
- Time stretch analog-to-digital converter
References
- J. R. Janesick (2001). Scientific charge-coupled devices. SPIE Press. ISBN 9780819436986.
- H. Zimmermann (2000). Integrated silicon optoelectronics. Springer. ISBN 978-3540666622.
- Chou, J.; Boyraz, O.; Solli, D.; Jalali, B. (2007). "Femtosecond real-time single-shot digitizer". Applied Physics Letters. 91 (16): 161105–1–161105–3. Bibcode:2007ApPhL..91p1105C. doi:10.1063/1.2799741 – via Researchgate.net.
- Solli, D.R.; Boyraz, O.; Jalali, B. (2008). "Amplified wavelength–time transformation for real-time spectroscopy". Nature Photonics. 2 (1): 48–51. Bibcode:2008NaPho...2...48S. doi:10.1038/nphoton.2007.253.
- K. Goda; K. K. Tsia & B. Jalali (2008). "Amplified dispersive Fourier-transform imaging for ultrafast displacement sensing and barcode reading". Applied Physics Letters. 93 (13): 131109. arXiv:0807.4967. Bibcode:2008ApPhL..93m1109G. doi:10.1063/1.2992064.
- K. Goda; K. K. Tsia & B. Jalali (2009). "Serial time-encoded amplified imaging for real-time observation of fast dynamic phenomena". Nature. 458 (7242): 1145–9. Bibcode:2009Natur.458.1145G. doi:10.1038/nature07980. PMID 19407796.
- A. Mahjoubfar; K. Goda; A. Ayazi; A. Fard; S. H. Kim & B. Jalali (2011). "High-speed nanometer-resolved imaging vibrometer and velocimeter". Applied Physics Letters. 98 (10): 101107. Bibcode:2011ApPhL..98j1107M. doi:10.1063/1.3563707.
- Chen, C.L.; Mahjoubfar, A.; Tai, L.; Blaby, I.; Huang, A.; Niazi, K.; Jalali, B. (2016). "Deep Learning in Label-free Cell Classification". Scientific Reports. 6: 21471. Bibcode:2016NatSR...621471C. doi:10.1038/srep21471. PMC 4791545. PMID 26975219.
- K. Goda; D. R. Solli; K. K. Tsia & B. Jalali (2009). "Theory of amplified dispersive Fourier transformation". Physical Review A. 80 (4): 043821. Bibcode:2009PhRvA..80d3821G. doi:10.1103/PhysRevA.80.043821. hdl:10722/91330.
- K. Goda & B. Jalali (2010). "Noise figure of amplified dispersive Fourier transformation". Physical Review A. 82 (3): 033827. Bibcode:2010PhRvA..82c3827G. doi:10.1103/PhysRevA.82.033827.
- Tsia K. K., Goda K., Capewell D., Jalali B. (2010). "Performance of serial time-encoded amplified microscope". Optics Express. 18 (10): 10016–28. Bibcode:2010OExpr..1810016T. doi:10.1364/oe.18.010016. hdl:10722/91333. PMID 20588855.CS1 maint: multiple names: authors list (link)
- Chen, Claire Lifan; Mahjoubfar, Ata; Tai, Li-Chia; Blaby, Ian K.; Huang, Allen; Niazi, Kayvan Reza; Jalali, Bahram (2016). "Deep Learning in Label-free Cell Classification". Scientific Reports. 6: 21471. Bibcode:2016NatSR...621471C. doi:10.1038/srep21471. PMC 4791545. PMID 26975219.published under CC BY 4.0 licensing
- Michaud, Sarah (5 April 2016). "Leveraging Big Data for Cell Imaging". Optics & Photonics News. The Optical Society. Retrieved 8 July 2016.
- "Photonic Time Stretch Microscopy Combined with Artificial Intelligence Spots Cancer Cells in Blood". Med Gadget. 15 April 2016. Retrieved 8 July 2016.
- Chen, Claire Lifan; Mahjoubfar, Ata; Jalali, Bahram (23 April 2015). "Optical Data Compression in Time Stretch Imaging". PLOS ONE. 10 (4): e0125106. doi:10.1371/journal.pone.0125106. PMC 4408077. PMID 25906244.
- Mahjoubfar, Ata; Churkin, Dmitry V.; Barland, Stéphane; Broderick, Neil; Turitsyn, Sergei K.; Jalali, Bahram (2017). "Time stretch and its applications". Nature Photonics. 11 (6): 341–351. Bibcode:2017NaPho..11..341M. doi:10.1038/nphoton.2017.76.
- G. Popescu, "Quantitative phase imaging of cells and tissues," McGraw Hill Professional (2011)
- Lau, Andy K. S.; Wong, Terence T. W.; Ho, Kenneth K. Y.; Tang, Matthew T. H.; Chan, Antony C. S.; Wei, Xiaoming; Lam, Edmund Y.; Shum, Ho Cheung; Wong, Kenneth K. Y.; Tsia, Kevin K. (2014). "Interferometric time-stretch microscopy for...quantitative cellular and tissue imaging" (PDF). Journal of Biomedical Optics. Free PDF download. 19 (7): 076001. Bibcode:2014JBO....19g6001L. doi:10.1117/1.JBO.19.7.076001. hdl:10722/200609. PMID 24983913.
- Chen C., Mahjoubfar A., Tai L., Blaby I., Huang A., Niazi K., Jalali B. (2016). "Deep Learning in Label-free Cell Classification". Scientific Reports. 6: 21471. Bibcode:2016NatSR...621471C. doi:10.1038/srep21471. PMC 4791545. PMID 26975219.CS1 maint: multiple names: authors list (link)
- D. Di Carlo (2009). "Inertial microfluidics". Lab on a Chip. 9 (21): 3038–46. doi:10.1039/b912547g. PMID 19823716.
- T. R. Hsu (2008). MEMS & microsystems: design, manufacture, and nanoscale engineering. Wiley. ISBN 978-0470083017.
- Mahjoubfar A., Goda K., Ayazi A., Fard A., Kim S., Jalali B. (2011). "High-speed nanometer-resolved imaging vibrometer and velocimeter". Applied Physics Letters. 98 (10): 101107. Bibcode:2011ApPhL..98j1107M. doi:10.1063/1.3563707.CS1 maint: multiple names: authors list (link)
- CL Chen; A Mahjoubfar; B Jalali (2015). "Optical Data Compression in Time Stretch Imaging". PLOS ONE. 10 (4): 1371. doi:10.1371/journal.pone.0125106. PMC 4408077. PMID 25906244.