Rigid line inclusion
A rigid line inclusion, also called stiffener, is a mathematical model used in solid mechanics to describe a narrow hard phase, dispersed within a matrix material. This inclusion is idealised as an infinitely rigid and thin reinforcement, so that it represents a sort of ‘inverse’ crack, from which the nomenclature ‘anticrack’ derives.
From the mechanical point of view, a stiffener introduces a kinematical constraint, imposing that it may only suffer a rigid body motion along its line.
Theoretical model
The stiffener model has been used to investigate different mechanical problems in classical elasticity (load diffusion,[1] inclusion at bi material interface [2]).
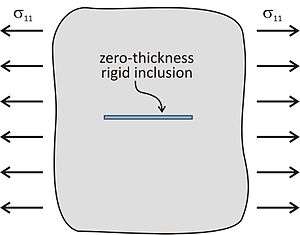
The main characteristics of the theoretical solutions are basically the following.
- Similarly to a fracture, a square-root singularity in the stress/strain fields is present at the tip of the inclusion.
- In a homogeneous matrix subject to uniform stress at infinity, such singularity only arises when a normal stress acts parallel or orthogonal to the inclusion line, while a stiffener parallel to a simple shear does not disturb the ambient field.
Experimental validation

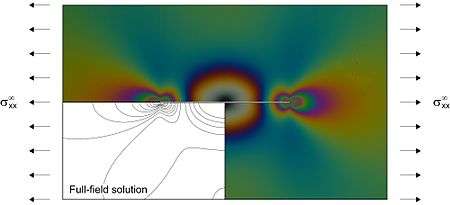
The characteristics of the elastic solution have been experimentally confirmed through photoelastic transmission experiments.[3]
Shear bands emerging at the stiffener tip
Analytical solutions obtained in prestressed elasticity show the possibility of the emergence of shear bands at the tip of the stiffener.[4][5][6][7]
References
- Koiter, W.T., On the diffusion of load from a stiffener into a sheet. Q. J. Mech. Appl. Math. 1955, VIII, 164–178.
- Ballarini, R., A rigid line inclusion at a bimaterial interface. Eng. Fract. Mech., 1990, 37, 1–5.
- G. Noselli, F. Dal Corso and D. Bigoni, The stress intensity near a stiffener disclosed by photoelasticity. International Journal of Fracture, 2010, 166, 91–103.
- Bigoni, D. Nonlinear Solid Mechanics: Bifurcation Theory and Material Instability. Cambridge University Press, 2012 . ISBN 9781107025417.
- F. Dal Corso, D. Bigoni and M. Gei, The stress concentration near a rigid line inclusion in a prestressed, elastic material. Part I Full-field solution and asymptotics. Journal of the Mechanics and Physics of Solids, 2008, 56, 815–838.
- D. Bigoni, F. Dal Corso and M. Gei, The stress concentration near a rigid line inclusion in a prestressed, elastic material. Part II Implications on shear band nucleation, growth and energy release rate. Journal of the Mechanics and Physics of Solids, 2008, 56, 839–857.
- F. Dal Corso and D. Bigoni, The interactions between shear bands and rigid lamellar inclusions in a ductile metal matrix. Proceedings of the Royal Society A, 2009, 465, 143–163.