Heart rate variability
Heart rate variability (HRV) is the physiological phenomenon of variation in the time interval between heartbeats. It is measured by the variation in the beat-to-beat interval.
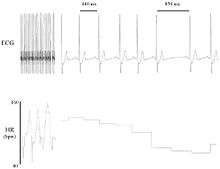
Other terms used include: "cycle length variability", "RR variability" (where R is a point corresponding to the peak of the QRS complex of the ECG wave; and RR is the interval between successive Rs), and "heart period variability".
Methods used to detect beats include: ECG, blood pressure, ballistocardiograms,[1][2] and the pulse wave signal derived from a photoplethysmograph (PPG). ECG is considered superior because it provides a clear waveform, which makes it easier to exclude heartbeats not originating in the sinoatrial node. The term "NN" is used in place of RR to emphasize the fact that the processed beats are "normal" beats.
Clinical significance
Reduced HRV has been shown to be a predictor of mortality after myocardial infarction[3][4] although others have shown that the information in HRV relevant to acute myocardial infarction survival is fully contained in the mean heart rate.[5] A range of other outcomes and conditions may also be associated with modified (usually lower) HRV, including congestive heart failure, diabetic neuropathy, post–cardiac-transplant depression, susceptibility to SIDS and poor survival in premature babies.
Psychological and social aspects
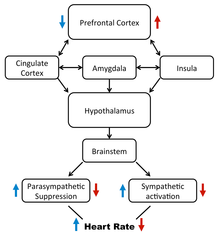
There is interest in HRV in the field of psychophysiology. For example, HRV is related to emotional arousal. High-frequency (HF) activity has been found to decrease under conditions of acute time pressure and emotional strain[7] and elevated anxiety state,[8] presumably related to focused attention and motor inhibition.[8] HRV has been shown to be reduced in individuals reporting to worry more.[9] In individuals with post-traumatic stress disorder (PTSD), HRV and its HF component (see below) is reduced whilst the low-frequency (LF) component is elevated. Furthermore, PTSD patients demonstrated no LF or HF reactivity to recalling a traumatic event.[10]
The neurovisceral integration is a model of HRV that views the central autonomic network as the decision maker of cognitive, behavioral and physiological regulation as they pertain to a continuum of emotion.[11] The neurovisceral integration model describes how the prefrontal cortex regulates activity in limbic structures which act to suppress parasympathetic activity and activate sympathetic circuits.[12] Variation in the output of these two branches of the autonomic system produces HRV[13] and activity in the prefrontal cortex can hence modulate HRV.[14]
HRV is the measure of the inconsistent gaps between each heartbeat and is used as an index for different aspects of psychology.[15] HRV is reported to be an index of the influence of both the parasympathetic nervous system and the sympathetic nervous systems.[16] Different aspects of psychology represent the balance of these two influences. For example, high HRV is shown proper emotion regulation, decision-making, and attention, and low HRV reflects the opposite.[16] The parasympathetic nervous system works quickly to decrease heart rate, while the SNS works slowly to increase heart rate, and this is important because it applies to the different psychological states mentioned above.[15] For example, someone with high HRV may reflect increased parasympathetic activity, and someone with low HRV may reflect increased sympathetic activity.[17]
Emotions stem from the time and impact of a situation on a person.[18] The ability to regulate emotions is essential for social environments and well-being.[15] HRV has provided a window to the physiological components associated with emotional regulation.[16] HRV has been shown to reflect emotional regulation at two different levels, while resting and while completing a task. Research suggests that a person with higher HRV while resting can provide more appropriate emotional responses compared to those that have low HRV at rest.[16] Empirical research found that HRV can reflect better emotional regulation by those with higher resting HRV, particularly with negative emotions.[19] When completing a task, HRV is subject to change, especially when people need to regulate their emotions. Most importantly, individual differences are related to the ability to regulate emotions.[20] Not only is emotional regulation necessary, but so is attention.
Previous research has suggested that a large part of the attention regulation is due to the default inhibitory properties of the prefrontal cortex.[16] Top-down processes from the prefrontal cortex provide parasympathetic influences, and if for some reason, those influences are active, attention can suffer.[16] For example, researchers have suggested that HRV can index attention. For example, a group of researchers found that groups with high anxiety and low HRV have poor attention.[21] In line with this research, it has also been suggested that increased attention has been linked to high HRV and increased vagus nerve activity.[16] The vagus nerve activity reflects the physiological modulation of the parasympathetic and sympathetic nervous system.[15] The activity behind the prefrontal cortex and the parasympathetic and sympathetic nervous system can influence heart activity. However, people are not all affected the same. A systematic review of HRV and cognitive function suggested that resting HRV can predict individual differences in attentional performance.[22] Even in psychological concepts such as attention, HRV can index individual differences. Furthermore, HRV has been able to index the role of attention and performance, supporting high HRV as a biomarker of increased attention and performance.[23] Both emotion and attention can shed light on how HRV is used as an index for decision making.
Decision-making skills are found to be indexed by HRV in several studies. Previous research has suggested that both emotion and attention are linked to decision making; for example, poor decision making is linked to the inability to regulate or control emotions and attention and vice versa.[21] Decision making is negatively affected by lower HRV and positively affected by higher levels of HRV. Most importantly, resting-state HRV was found to be a significant predictor of cognitive functions such as decision making.[22] HRV, accompanied by a psychological state, such as anxiety, has been found to lead to poor decisions. For example, a group of researchers found that low HRV was an index of higher uncertainty leading to poor decision-making skills, especially those with higher levels of anxiety.[21] HRV was also used to assess decision-making skills in a high-risk game and was found to be an index higher sympathetic activation (lower HRV) when making decisions involving risk.[24] HRV can index psychological concepts, such as the ones outlined above, to assess the demand for the situations that people experience.
The polyvagal theory[25][26] is another way to describe the pathways in the autonomic nervous system that mediate HRV. The polyvagal theory highlights three main ordinal processes, inactive response to an environmental threat, the active response to an environmental threat, and the fluctuation between the connect and disconnect to an environmental threat.[11] This theory decomposes heart rate variability based on frequency domain characteristics with an emphasis on respiratory sinus arrhythmia and its transmission by a neural pathway that is distinct from other components of HRV.[27] There is anatomic[28] and physiological[29] evidence for a polyvagal control of the heart.
Variation
Variation in the beat-to-beat interval is a physiological phenomenon. The SA node receives several different inputs and the instantaneous heart rate or RR interval and its variation are the results of these inputs.
The main inputs are the sympathetic and the parasympathetic nervous system (PSNS) and humoral factors. Respiration gives rise to waves in heart rate mediated primarily via the PSNS, and it is thought that the lag in the baroreceptor feedback loop may give rise to 10 second waves in heart rate (associated with Mayer waves of blood pressure), but this remains controversial.
Factors that affect the input are the baroreflex, thermoregulation, hormones, sleep-wake cycle, meals, physical activity, and stress.
Decreased PSNS activity or increased SNS activity will result in reduced HRV. High frequency (HF) activity (0.15 to 0.40 Hz), especially, has been linked to PSNS activity. Activity in this range is associated with the respiratory sinus arrhythmia (RSA), a vagally mediated modulation of heart rate such that it increases during inspiration and decreases during expiration. Less is known about the physiological inputs of the low frequency (LF) activity (0.04 to 0.15 Hz). Though previously thought to reflect SNS activity, it is now widely accepted that it reflects a mixture of both the SNS and PSNS.[30]
Phenomena
There are two primary fluctuations:
- Respiratory arrhythmia (or respiratory sinus arrhythmia).[31][32] This heart rate variation is associated with respiration and faithfully tracks the respiratory rate across a range of frequencies.
- Low-frequency oscillations.[33] This heart rate variation is associated with Mayer waves (Traube–Hering–Mayer waves) of blood pressure and is usually at a frequency of 0.1 Hz, or a 10-second period.
Artifact
Errors in the location of the instantaneous heart beat will result in errors in the calculation of the HRV. HRV is highly sensitive to artifact and errors in as low as even 2% of the data will result in unwanted biases in HRV calculations. To ensure accurate results therefore it is critical to manage artifact and RR errors appropriately prior to performing any HRV analyses.[34][35]
Robust management of artifacts, including RWave identification, interpolation and exclusion requires a high degree of care and precision. This can be very time consuming in large studies with data recorded over long durations. Software packages are able to assist users with a variety of robust and tested artifact management tools. These software programs also include some automated capability but it is important that a human review any automated artifact management and edit accordingly.
Analysis
The most widely used methods can be grouped under time-domain and frequency-domain. A joint European and American task-force described standards in HRV measurements in 1996.[13] Other methods have been proposed, such as non-linear methods.
Time-domain methods[36]
These are based on the beat-to-beat or NN intervals, which are analysed to give variables such as:[36]
- SDNN, the standard deviation of NN intervals. Often calculated over a 24-hour period. SDANN, the standard deviation of the average NN intervals calculated over short periods, usually 5 minutes. SDNN is therefore a measure of changes in heart rate due to cycles longer than 5 minutes. SDNN reflects all the cyclic components responsible for variability in the period of recording, therefore it represents total variability.
- RMSSD ("root mean square of successive differences"), the square root of the mean of the squares of the successive differences between adjacent NNs.[36]
- SDSD ("standard deviation of successive differences"), the standard deviation of the successive differences between adjacent NNs.[36]
- NN50, the number of pairs of successive NNs that differ by more than 50 ms.
- pNN50, the proportion of NN50 divided by total number of NNs.
- NN20, the number of pairs of successive NNs that differ by more than 20 ms.[37]
- pNN20, the proportion of NN20 divided by total number of NNs.
- EBC ("estimated breath cycle"), the range (max-min) within a moving window of a given time duration within the study period. The windows can move in a self-overlapping way or be strictly distinct (sequential) windows. EBC is often provided in data acquisition scenarios where HRV feedback in real time is a primary goal. EBC derived from PPG over 10-second and 16-second sequential and overlapping windows has been shown to correlate highly with SDNN.[38]
Geometric methods
The series of NN intervals also can be converted into a geometric pattern such as: Geometric Measures HRV triangular index: integral of density distribution / maximum of density distribution maximum HRV triangular index = Number of all NN intervals / maximum number. Dependent on the length of the bin -> quote the bin size+ relative insensitive to the analytic quality of the series of NN intervals - need of reasonable number of NN intervals to generate the geometric pattern (in practice 20 min to 24 h)-not appropriate to assess short-term changes in HRV
- the sample density distribution of NN interval durations;
- sample density distribution of differences between adjacent NN intervals;
- a scatterplot of each NN (or RR) interval with the immediately preceding NN (or RR) interval [39] — also called "Poincare plot" or (apparently in error [40]) a "Lorenz plot";
and so forth. A simple formula is then used that judges the variability on the basis of the geometric and/or graphics properties of the resulting pattern.
Frequency-domain methods[36]
Frequency domain methods assign bands of frequency and then count the number of NN intervals that match each band. The bands are typically high frequency (HF) from 0.15 to 0.4 Hz, low frequency (LF) from 0.04 to 0.15 Hz, and the very low frequency (VLF) from 0.0033 to 0.04 Hz.
Several methods of analysis are available. Power spectral density (PSD), using parametric or nonparametric methods, provides basic information on the power distribution across frequencies. One of the most commonly used PSD methods is the discrete Fourier transform. Methods for the calculation of PSD may be generally classified as nonparametric and parametric. In most instances, both methods provide comparable results. The advantages of the nonparametric methods are (1) the simplicity of the algorithm used (fast Fourier transform [FFT] in most of the cases) and (2) the high processing speed. The advantages of parametric methods are (1) smoother spectral components that can be distinguished independent of preselected frequency bands, (2) easy postprocessing of the spectrum with an automatic calculation of low- and high-frequency power components with an easy identification of the central frequency of each component, and (3) an accurate estimation of PSD even on a small number of samples on which the signal is supposed to maintain stationarity. The basic disadvantage of parametric methods is the need of verification of the suitability of the chosen model and of its complexity (that is, the order of the model).
In addition to classical FFT-based methods used for the calculation of frequency parameters, a more appropriate PSD estimation method is the Lomb–Scargle periodogram.[41] Analysis has shown that the LS periodogram can produce a more accurate estimate of the PSD than FFT methods for typical RR data. Since the RR data is an unevenly sampled data, another advantage of the LS method is that in contrast to FFT-based methods it is able to be used without the need to resample and detrend the RR data.
Alternatively, to avoid artefacts that are created when calculating the power of a signal that includes a single high-intensity peak (for example caused by an arrhythmic heart beat), the concept of the 'instantaneous Amplitude' has been introduced, which is based on the Hilbert transform of the RR data.[42]
A newly used HRV index, which depends on the wavelet entropy measures, is an alternative choice. The wavelet entropy measures are calculated using a three-step procedure defined in the literature. First, the wavelet packet algorithm is implemented using the Daubechies 4 (DB4) function as the mother wavelet with a scale of 7. Once the wavelet coefficients are obtained, the energy for each coefficient are calculated as described in the literature. After calculating the normalized values of wavelet energies, which represent the relative wavelet energy (or the probability distribution), the wavelet entropies are obtained using the definition of entropy given by Shannon.
Non-linear methods
Given the complexity of the mechanisms regulating heart rate, it is reasonable to assume that applying HRV analysis based on methods of non-linear dynamics will yield valuable information. Although chaotic behavior has been assumed, more rigorous testing has shown that heart rate variability cannot be described as a low dimensional chaotic process.[43] However, application of chaotic globals to HRV has been shown to predict diabetes status.[44] The most commonly used non-linear method of analysing heart rate variability is the Poincaré plot. Each data point represents a pair of successive beats, the x-axis is the current RR interval, while the y-axis is the previous RR interval. HRV is quantified by fitting mathematically defined geometric shapes to the data.[45] Other methods used are the correlation dimension, symbolic dynamics,[46] nonlinear predictability,[43] pointwise correlation dimension,[47] detrended fluctuation analysis,[48][49] approximate entropy, sample entropy,[50] multiscale entropy analysis,[51] sample asymmetry[52] and memory length (based on inverse statistical analysis).[53][54] It is also possible to represent long range correlations geometrically.[55]
Long term correlations
Sequences of RR intervals have been found to have long-term correlations.[56][55] However, one flaw with these analyses is their lack of goodness-of-fit statistics, i.e. values are derived that may or may not have adequate statistical rigor. Different types of correlations have been found during different sleep stages.[57][55]
Cross correlation with other systems
The question of how heartbeat rhythms are correlated with other physiologic systems such as lung and brain was studied by Bashan et al[58] . It is found that while during wake, light and REM sleep the correlation between heartbeat with other physiological systems are high, they almost vanish during deep sleep.
Duration and circumstances of ECG recording
Time domain methods are preferred to frequency domain methods when short-term recordings are investigated. This is due to the fact that the recording should be at least 10 times the wavelength of the lowest frequency bound of interest. Thus, recording of approximately 1 minute is needed to assess the HF components of HRV (i.e., a lowest bound of 0.15 Hz is a cycle of 6.6 seconds and so 10 cycles require ~60 seconds), while more than 4 minutes are needed to address the LF component (with a lower bound of 0.04 Hz).
Although time domain methods, especially the SDNN and RMSSD methods, can be used to investigate recordings of long durations, a substantial part of the long-term variability is day–night differences. Thus, long-term recordings analyzed by time domain methods should contain at least 18 hours of analyzable ECG data that include the whole night.
Physiological correlates of HRV components
Autonomic influences of heart rate
Although cardiac automaticity is intrinsic to various pacemaker tissues, heart rate and rhythm are largely under the control of the autonomic nervous system. The parasympathetic influence on heart rate is mediated via release of acetylcholine by the vagus nerve. Muscarinic acetylcholine receptors respond to this release mostly by an increase in cell membrane K+ conductance. Acetylcholine also inhibits the hyperpolarization-activated "pacemaker" current. The "Ik decay" hypothesis proposes that pacemaker depolarization results from slow deactivation of the delayed rectifier current, Ik, which, due to a time-independent background inward current, causes diastolic depolarization. Conversely, the "If activation" hypothesis suggests that after action potential termination, If provides a slowly activating inward current predominating over decaying Ik, thus initiating slow diastolic depolarization.
The sympathetic influence on heart rate is mediated by release of epinephrine and norepinephrine. Activation of β-adrenergic receptors results in cAMP-mediated phosphorylation of membrane proteins and increases in ICaL and in If the end result is an acceleration of the slow diastolic depolarization.
Under resting conditions, vagal tone prevails and variations in heart period are largely dependent on vagal modulation. The vagal and sympathetic activity constantly interact. Because the sinus node is rich in acetylcholinesterase, the effect of any vagal impulse is brief because the acetylcholine is rapidly hydrolyzed. Parasympathetic influences exceed sympathetic effects probably through two independent mechanisms: a cholinergically induced reduction of norepinephrine released in response to sympathetic activity, and a cholinergic attenuation of the response to an adrenergic stimulus.
Components
The RR interval variations present during resting conditions represent beat-by-beat variations in cardiac autonomic inputs. However, efferent vagal (parasympathetic) activity is a major contributor to the HF component, as seen in clinical and experimental observations of autonomic maneuvers such as electrical vagal stimulation, muscarinic receptor blockade, and vagotomy. More problematic is the interpretation of the LF component, which was considered by some as a marker of sympathetic modulation (especially when expressed in normalized units) but is now known to include both sympathetic and vagal influences. For example, during sympathetic activation the resulting tachycardia is usually accompanied by a marked reduction in total power, whereas the reverse occurs during vagal activation. Thus the spectral components change in the same direction and do not indicate that LF faithfully reflects sympathetic effects.
HRV measures fluctuations in autonomic inputs to the heart rather than the mean level of autonomic inputs. Thus, both withdrawal and saturatingly high levels of autonomic input to the heart can lead to diminished HRV.
Changes related to specific pathologies
A reduction of HRV has been reported in several cardiovascular and noncardiovascular diseases.
Myocardial infarction
Depressed HRV after MI may reflect a decrease in vagal activity directed to the heart. HRV in patients surviving an acute MI reveal a reduction in total and in the individual power of spectral components. The presence of an alteration in neural control is also reflected in a blunting of day-night variations of RR interval. In post-MI patients with a very depressed HRV, most of the residual energy is distributed in the VLF frequency range below 0.03 Hz, with only a small respiration-related variations.
Diabetic neuropathy
In neuropathy associated with diabetes mellitus characterized by alteration in small nerve fibers, a reduction in time domain parameters of HRV seems not only to carry negative prognostic value but also to precede the clinical expression of autonomic neuropathy. In diabetic patients without evidence of autonomic neuropathy, reduction of the absolute power of LF and HF during controlled conditions was also reported. Similarly, diabetic patients can be differentiated from normal controls on the basis of reduction in HRV.[44]
Cardiac transplantation
A very reduced HRV with no definite spectral components has been reported in patients with a recent heart transplant. The appearance of discrete spectral components in a few patients is considered to reflect cardiac reinnervation. This reinnervation may occur as early as 1 to 2 years after transplantation and is assumed to be of sympathetic origin. In addition, a correlation between respiratory rate and the HF component of HRV observed in some transplanted patients also indicates that a nonneural mechanism may generate a respiration-related rhythmic oscillation.
Myocardial dysfunction
A reduced HRV has been observed consistently in patients with cardiac failure. In this condition characterized by signs of sympathetic activation such as faster heart rates and high levels of circulating catecholamines, a relation between changes in HRV and the extent of left ventricular dysfunction was reported. In fact, whereas the reduction in time domain measures of HRV seemed to parallel the severity of the disease, the relationship between spectral components and indices of ventricular dysfunction appears to be more complex. In particular, in most patients with a very advanced phase of the disease and with a drastic reduction in HRV, an LF component could not be detected despite the clinical signs of sympathetic activation. This reflects that, as stated above, the LF may not accurately reflect cardiac sympathetic tone.
Liver cirrhosis
Liver cirrhosis is associated with decreased HRV. Decreased HRV in patients with cirrhosis has a prognostic value and predicts mortality. Loss of HRV is also associated with higher plasma pro-inflammatory cytokine levels and impaired neurocognitive function in this patient population.[59]
Sepsis
HRV is decreased in patients with sepsis. Loss of HRV has both diagnostic and prognostic value in neonates with sepsis.[60] The pathophysiology of decreased HRV in sepsis is not well understood but there is experimental evidence to show that partial uncoupling of cardiac pacemaker cells from autonomic neural control may play a role in decreased HRV during acute systemic inflammation.[61]
Tetraplegia
Patients with chronic complete high cervical spinal cord lesions have intact efferent vagal neural pathways directed to the sinus node. However, an LF component can be detected in HRV and arterial pressure variabilities of some tetraplegic patients. Thus, the LF component of HRV in those without intact sympathetic inputs to the heart represent vagal modulation.
Sudden cardiac death
Victims of sudden cardiac death have been found to have had lower HRV than healthy individuals.[62][55]
Cancer
HRV correlates with the progression of disease and outcome of cancer patients, according to a systematic review of published studies.[63]
Modifications by specific interventions
Interventions that augment HRV may be protective against cardiac mortality and sudden cardiac death. Although the rationale for changing HRV is sound, it also contains the inherent danger of leading to the unwarranted assumption that modification of HRV translates directly into cardiac protection, which may not be the case. Despite the growing consensus that increases in vagal activity can be beneficial, it is not as yet known how much vagal activity (or HRV as a marker) has to increase in order to provide adequate protection.
β-Adrenergic blockade
The data on the effect of β-blockers on HRV in post-MI patients are surprisingly scant. Despite the observation of statistically significant increases, the actual changes are very modest. In conscious post-MI dogs, β-blockers do not modify HRV. The unexpected observation that before MI, β-blockade increases HRV only in the animals destined to be at low risk for lethal arrhythmias after MI may suggest novel approaches to post-MI risk stratification.
Antiarrhythmic drugs
Data exist for several antiarrhythmic drugs. Flecainide and propafenone but not amiodarone were reported to decrease time domain measures of HRV in patients with chronic ventricular arrhythmia. In another study, propafenone reduced HRV and decreased LF much more than HF. A larger study confirmed that flecainide, also encainide and moricizine, decreased HRV in post-MI patients but found no correlation between the change in HRV and mortality during follow-up. Thus, some antiarrhythmic drugs associated with increased mortality can reduce HRV. However, it is not known whether these changes in HRV have any direct prognostic significance.
Scopolamine
Low-dose muscarinic receptor blockers, such as atropine and scopolamine, may produce a paradoxical increase in vagal effects on the heart, as suggested by a decrease in heart rate. In addition, scopolamine and low dose atropine can markedly increase HRV. However, though the heart rate slowing in proportional to the (low) dose of atropine, the increase in HRV varies widely across and within individuals. This suggests that even for vagal activity to the heart, HRV may be a limited marker.
Thrombolysis
The effect of thrombolysis on HRV (assessed by pNN50) was reported in 95 patients with acute MI. HRV was higher 90 minutes after thrombolysis in the patients with patency of the infarct-related artery. However, this difference was no longer evident when the entire 24 hours were analyzed.
Exercise training
Exercise training may decrease cardiovascular mortality and sudden cardiac death. Regular exercise training is also thought to modify cardiac autonomic control. Individuals who exercise regularly have a 'training bradycardia' (i.e., low resting heart rate) and generally have higher HRV than sedentary individuals.
Biofeedback
The technique called resonant breathing biofeedback teaches how to recognize and control involuntary heart rate variability. A randomized study by Sutarto et al. assessed the effect of resonant breathing biofeedback among manufacturing operators; depression, anxiety and stress significantly decreased.[64] A first overall meta-analysis by Goessl VC et al. (24 studies, 484 individuals, 2017) indicates ''HRV biofeedback training is associated with a large reduction in self-reported stress and anxiety'', while mentioning that more well-controlled studies are needed.[65]
Wind instruments
One study that surveyed the physiological effects of playing Native American flutes found a significant HRV increase when playing both low-pitched and high-pitched flutes.[66]
Normal values of standard measures
Even though there are no widely accepted standard values for HRV that can be used for clinical purposes, The Task Force of the European Society of Cardiology and Heart Rhythm Society (formerly called North American Society of Pacing Electrophysiology) provided initial normative values of standard measures of HRV[67]
See also
References
- Brüser C, Stadlthanner K, de Waele S, Leonhardt S (September 2011). "Adaptive beat-to-beat heart rate estimation in ballistocardiograms". IEEE Transactions on Information Technology in Biomedicine. 15 (5): 778–86. doi:10.1109/TITB.2011.2128337. PMID 21421447.
- Brüser C, Winter S, Leonhardt S (2012). "Unsupervised Heart Rate Variability Estimation from Ballistocardiograms". 7th International Workshop on Biosignal Interpretation (BSI 2012), Como, Italy.
- Bigger JT, Fleiss JL, Steinman RC, Rolnitzky LM, Kleiger RE, Rottman JN (January 1992). "Frequency domain measures of heart period variability and mortality after myocardial infarction". Circulation. 85 (1): 164–71. doi:10.1161/01.CIR.85.1.164. PMID 1728446.
- Kleiger RE, Miller JP, Bigger JT, Moss AJ (February 1987). "Decreased heart rate variability and its association with increased mortality after acute myocardial infarction". The American Journal of Cardiology. 59 (4): 256–62. doi:10.1016/0002-9149(87)90795-8. PMID 3812275.
- Abildstrom SZ, Jensen BT, Agner E, Torp-Pedersen C, Nyvad O, Wachtell K, Ottesen MM, Kanters JK (February 2003). "Heart rate versus heart rate variability in risk prediction after myocardial infarction". Journal of Cardiovascular Electrophysiology. 14 (2): 168–73. doi:10.1046/j.1540-8167.2003.02367.x. PMID 12693499.
- Nikolin S, Boonstra TW, Loo CK, Martin D (2017-08-03). "Combined effect of prefrontal transcranial direct current stimulation and a working memory task on heart rate variability". PLOS ONE. 12 (8): e0181833. Bibcode:2017PLoSO..1281833N. doi:10.1371/journal.pone.0181833. PMC 5542548. PMID 28771509.
- Nickel P, Nachreiner F (2003). "Sensitivity and diagnosticity of the 0.1-Hz component of heart rate variability as an indicator of mental workload". Human Factors. 45 (4): 575–90. doi:10.1518/hfes.45.4.575.27094. PMID 15055455.
- Jönsson P (January 2007). "Respiratory sinus arrhythmia as a function of state anxiety in healthy individuals". International Journal of Psychophysiology. 63 (1): 48–54. doi:10.1016/j.ijpsycho.2006.08.002. PMID 16989914.
- Brosschot JF, Van Dijk E, Thayer JF (January 2007). "Daily worry is related to low heart rate variability during waking and the subsequent nocturnal sleep period". International Journal of Psychophysiology. 63 (1): 39–47. doi:10.1016/j.ijpsycho.2006.07.016. PMID 17020787.
- Cohen H, Kotler M, Matar MA, Kaplan Z, Loewenthal U, Miodownik H, Cassuto Y (November 1998). "Analysis of heart rate variability in posttraumatic stress disorder patients in response to a trauma-related reminder". Biological Psychiatry. 44 (10): 1054–9. doi:10.1016/S0006-3223(97)00475-7. PMID 9821570.
- Appelhans, Bradley M.; Luecken, Linda J. (September 2006). "Heart Rate Variability as an Index of Regulated Emotional Responding". Review of General Psychology. 10 (3): 229–240. doi:10.1037/1089-2680.10.3.229. ISSN 1089-2680.
- Thayer JF, Sternberg E (November 2006). "Beyond heart rate variability: vagal regulation of allostatic systems". Annals of the New York Academy of Sciences. 1088 (1): 361–72. Bibcode:2006NYASA1088..361T. doi:10.1196/annals.1366.014. PMID 17192580.
- Task Force of the European Society of Cardiology and the North American Society of Pacing and Electrophysiology (March 1996). "Heart rate variability. Standards of measurement, physiological interpretation, and clinical use". European Heart Journal. 17 (3): 354–81. doi:10.1093/oxfordjournals.eurheartj.a014868. PMID 8737210.
- Napadow V, Dhond R, Conti G, Makris N, Brown EN, Barbieri R (August 2008). "Brain correlates of autonomic modulation: combining heart rate variability with fMRI". NeuroImage. 42 (1): 169–77. doi:10.1016/j.neuroimage.2008.04.238. PMC 2603289. PMID 18524629.
- Appelhans, Bradley M.; Luecken, Linda J. (September 2006). "Heart Rate Variability as an Index of Regulated Emotional Responding". Review of General Psychology. 10 (3): 229–240. doi:10.1037/1089-2680.10.3.229. ISSN 1089-2680.
- Thayer, Julian F.; Lane, Richard D. (February 2009). "Claude Bernard and the heart–brain connection: Further elaboration of a model of neurovisceral integration". Neuroscience & Biobehavioral Reviews. 33 (2): 81–88. doi:10.1016/j.neubiorev.2008.08.004. ISSN 0149-7634. PMID 18771686.
- Laborde, Sylvain; Mosley, Emma; Thayer, Julian F. (2017-02-20). "Heart Rate Variability and Cardiac Vagal Tone in Psychophysiological Research – Recommendations for Experiment Planning, Data Analysis, and Data Reporting". Frontiers in Psychology. 08: 213. doi:10.3389/fpsyg.2017.00213. ISSN 1664-1078. PMC 5316555. PMID 28265249.
- Thayer, Julian F; Lane, Richard D (December 2000). "A model of neurovisceral integration in emotion regulation and dysregulation". Journal of Affective Disorders. 61 (3): 201–216. doi:10.1016/s0165-0327(00)00338-4. ISSN 0165-0327. PMID 11163422.
- Choi, Kwang-Ho; Kim, Junbeom; Kwon, O. Sang; Kim, Min Ji; Ryu, Yeon Hee; Park, Ji-Eun (May 2017). "Is heart rate variability (HRV) an adequate tool for evaluating human emotions? – A focus on the use of the International Affective Picture System (IAPS)". Psychiatry Research. 251: 192–196. doi:10.1016/j.psychres.2017.02.025. ISSN 0165-1781. PMID 28213189.
- Park, Gewnhi; Thayer, Julian F. (2014-05-01). "From the heart to the mind: cardiac vagal tone modulates top-down and bottom-up visual perception and attention to emotional stimuli". Frontiers in Psychology. 5: 278. doi:10.3389/fpsyg.2014.00278. ISSN 1664-1078. PMC 4013470. PMID 24817853.
- Ramírez, Encarnación; Ortega, Ana Raquel; Reyes Del Paso, Gustavo A. (December 2015). "Anxiety, attention, and decision making: The moderating role of heart rate variability". International Journal of Psychophysiology. 98 (3): 490–496. doi:10.1016/j.ijpsycho.2015.10.007. ISSN 0167-8760. PMID 26555079.
- Forte, Giuseppe; Favieri, Francesca; Casagrande, Maria (2019-07-09). "Heart Rate Variability and Cognitive Function: A Systematic Review". Frontiers in Neuroscience. 13: 710. doi:10.3389/fnins.2019.00710. ISSN 1662-453X. PMC 6637318. PMID 31354419.
- Colzato, Lorenza S.; Steenbergen, Laura (2017). "High vagally mediated resting-state heart rate variability is associated with superior action cascading". Neuropsychologia. 106: 1–6. doi:10.1016/j.neuropsychologia.2017.08.030. OCLC 1051786844. PMID 28866318.
- Shapiro, Martin S.; Rylant, Rhanda; de Lima, Amanda; Vidaurri, Andrea; van de Werfhorst, Herman (October 2017). "Playing a rigged game: Inequality's effect on physiological stress responses". Physiology & Behavior. 180: 60–69. doi:10.1016/j.physbeh.2017.08.006. ISSN 0031-9384. PMID 28818539.
- Porges SW (August 2003). "The Polyvagal Theory: phylogenetic contributions to social behavior". Physiology & Behavior. 79 (3): 503–13. doi:10.1016/S0031-9384(03)00156-2. PMID 12954445.
- Porges, Stephen W. (2011-04-25). The polyvagal theory : neurophysiological foundations of emotions, attachment, communication, and self-regulation (1st ed.). New York: W. W. Norton. ISBN 978-0393707007.
- Porges SW (February 2007). "The polyvagal perspective". Biological Psychology. 74 (2): 116–43. doi:10.1016/j.biopsycho.2006.06.009. PMC 1868418. PMID 17049418.
- Haselton JR, Solomon IC, Motekaitis AM, Kaufman MP (September 1992). "Bronchomotor vagal preganglionic cell bodies in the dog: an anatomic and functional study". Journal of Applied Physiology. 73 (3): 1122–9. doi:10.1152/jappl.1992.73.3.1122. PMID 1400025.
- Gatti PJ, Johnson TA, Massari VJ (February 1996). "Can neurons in the nucleus ambiguus selectively regulate cardiac rate and atrio-ventricular conduction?". Journal of the Autonomic Nervous System. 57 (1–2): 123–7. doi:10.1016/0165-1838(95)00104-2. PMID 8867095.
- Billman GE (2013). "The LF/HF ratio does not accurately measure cardiac sympatho-vagal balance". Frontiers in Physiology. 4: 26. doi:10.3389/fphys.2013.00026. PMC 3576706. PMID 23431279.
- Hales S (1733). Statistical Essays: Containing Haemastaticks. London, UK: Innys, Manby and Woodward.
- von Haller A. Elementa Physiologica. Lausanne, Switzerland: 1760; T II, Lit VI, 330
- Sayers BM (January 1973). "Analysis of heart rate variability". Ergonomics. 16 (1): 17–32. doi:10.1080/00140137308924479. PMID 4702060.
- Citi L, Brown EN, Barbieri R (2012). "Online tool for the detection and correction of erroneous and ectopic heartbeats".
- Citi L, Brown EN, Barbieri R (October 2012). "A real-time automated point-process method for the detection and correction of erroneous and ectopic heartbeats". IEEE Transactions on Bio-Medical Engineering. 59 (10): 2828–37. doi:10.1109/TBME.2012.2211356. PMC 3523127. PMID 22875239.
- Golgouneh, Alireza; Tarvirdizadeh, Bahram (2019-06-07). "Fabrication of a portable device for stress monitoring using wearable sensors and soft computing algorithms". Neural Computing and Applications. doi:10.1007/s00521-019-04278-7. ISSN 0941-0643.
- Mietus JE, Peng CK, Henry I, Goldsmith RL, Goldberger AL (October 2002). "The pNNx files: re-examining a widely used heart rate variability measure". Heart. 88 (4): 378–80. doi:10.1136/heart.88.4.378. PMC 1767394. PMID 12231596.
-
Goss CF, Miller EB (August 2013). "Dynamic Metrics of Heart Rate Variability". arXiv:1308.6018. Bibcode:2013arXiv1308.6018G. Cite journal requires
|journal=
(help) - Parameter aus dem Lorenz-Plot (Parameters from the Lorenz-Plot brain & heart, "Parameter der Herzratenvariabilität" (Parameters of HRV), by Dr. Egon Winter, Austria. Accessed 2016-11-20. In German.
- 'LORENZ CURVE' command in online user manual for 'Dataplot' software, published by NIST, U.S.A. Accessed via Version cached by Google on 2016-10-28 on 2016-11-20.
- Işler Y, Kuntalp M (October 2007). "Combining classical HRV indices with wavelet entropy measures improves to performance in diagnosing congestive heart failure". Computers in Biology and Medicine. 37 (10): 1502–10. doi:10.1016/j.compbiomed.2007.01.012. PMID 17359959.
- von Rosenberg W, Chanwimalueang T, Adjei T, Jaffer U, Goverdovsky V, Mandic DP (2017). "Resolving Ambiguities in the LF/HF Ratio: LF-HF Scatter Plots for the Categorization of Mental and Physical Stress from HRV". Frontiers in Physiology. 8: 360. doi:10.3389/fphys.2017.00360. PMC 5469891. PMID 28659811.
- Kanters JK, Holstein-Rathlou NH, Agner E (July 1994). "Lack of evidence for low-dimensional chaos in heart rate variability". Journal of Cardiovascular Electrophysiology. 5 (7): 591–601. doi:10.1111/j.1540-8167.1994.tb01300.x. PMID 7987529.
- De Souza NM, Vanderlei LC, Garner DM (2 January 2015). "Risk evaluation of diabetes mellitus by relation of chaotic globals to HRV". Complexity. 20 (3): 84–92. Bibcode:2015Cmplx..20c..84D. doi:10.1002/cplx.21508.
- Brennan M; Palaniswami M; Kamen P (2001). "Do existing measures of Poincaré plot geometry reflect non-linear features of heart rate variability? Biomedical Engineering, IEEE Transactions on, Proc". IEEE Transactions on Biomedical Engineering. 48 (11): 1342–1347. doi:10.1109/10.959330. PMID 11686633.
- Voss A, Schulz S, Schroeder R, Baumert M, Caminal P (January 2009). "Methods derived from nonlinear dynamics for analysing heart rate variability". Philosophical Transactions. Series A, Mathematical, Physical, and Engineering Sciences. 367 (1887): 277–96. Bibcode:2009RSPTA.367..277V. doi:10.1098/rsta.2008.0232. PMID 18977726.
- Storella RJ, Wood HW, Mills KM, Kanters JK, Højgaard MV, Holstein-Rathlou NH (October 1998). "Approximate entropy and point correlation dimension of heart rate variability in healthy subjects". Integrative Physiological and Behavioral Science. 33 (4): 315–20. doi:10.1007/BF02688699. PMID 10333974.
- Kantelhardt JW, Koscielny-Bunde E, Rego HH, Havlin S, Bunde A (2001). "Detecting long-range correlations with detrended fluctuation analysis". Physica A: Statistical Mechanics and Its Applications. 295 (3–4): 441–454. arXiv:cond-mat/0102214. Bibcode:2001PhyA..295..441K. doi:10.1016/S0378-4371(01)00144-3. ISSN 0378-4371.
- Peng CK, Havlin S, Stanley HE, Goldberger AL (1995). "Quantification of scaling exponents and crossover phenomena in nonstationary heartbeat time series". Chaos. 5 (1): 82–7. Bibcode:1995Chaos...5...82P. doi:10.1063/1.166141. PMID 11538314.
- Richman JS, Moorman JR (June 2000). "Physiological time-series analysis using approximate entropy and sample entropy". American Journal of Physiology. Heart and Circulatory Physiology. 278 (6): H2039–49. doi:10.1152/ajpheart.2000.278.6.H2039. PMID 10843903.
- Costa M, Goldberger AL, Peng CK (August 2002). "Multiscale entropy analysis of complex physiologic time series" (PDF). Physical Review Letters. 89 (6): 068102. Bibcode:2002PhRvL..89f8102C. doi:10.1103/physrevlett.89.068102. PMID 12190613.
- Kovatchev BP, Farhy LS, Cao H, Griffin MP, Lake DE, Moorman JR (December 2003). "Sample asymmetry analysis of heart rate characteristics with application to neonatal sepsis and systemic inflammatory response syndrome". Pediatric Research. 54 (6): 892–8. doi:10.1203/01.pdr.0000088074.97781.4f. PMID 12930915.
- Shirazi AH, Raoufy MR, Ebadi H, De Rui M, Schiff S, Mazloom R, Hajizadeh S, Gharibzadeh S, Dehpour AR, Amodio P, Jafari GR, Montagnese S, Mani AR (2013). "Quantifying memory in complex physiological time-series". PLOS ONE. 8 (9): e72854. Bibcode:2013PLoSO...872854S. doi:10.1371/journal.pone.0072854. PMC 3764113. PMID 24039811.
- Ebadi H, Shirazi AH, Mani AR, Jafari GR (24 August 2011). "Inverse statistical approach in heartbeat time series". Journal of Statistical Mechanics: Theory and Experiment. 2011 (8): P08014. Bibcode:2011JSMTE..08..014E. doi:10.1088/1742-5468/2011/08/P08014.
- Bailly F, Longo G, Montevil M (September 2011). "A 2-dimensional geometry for biological time". Progress in Biophysics and Molecular Biology. 106 (3): 474–84. arXiv:1004.4186. doi:10.1016/j.pbiomolbio.2011.02.001. PMID 21316386.
- Mietus J, Hausdorff JM, Havlin S, Stanley HE, Goldberger AL (March 1993). "Long-range anticorrelations and non-Gaussian behavior of the heartbeat". Physical Review Letters. 70 (9): 1343–6. Bibcode:1993PhRvL..70.1343P. doi:10.1103/PhysRevLett.70.1343. PMID 10054352.
- Bunde A, Havlin S, Kantelhardt JW, Penzel T, Peter JH, Voigt K (October 2000). "Correlated and uncorrelated regions in heart-rate fluctuations during sleep". Physical Review Letters. 85 (17): 3736–9. Bibcode:2000PhRvL..85.3736B. doi:10.1103/PhysRevLett.85.3736. PMID 11030994.
- A. Bashan; R.P. Bartsch; J.W. Kantelhardt; S. Havlin; P.C. Ivanov (2012). "Network physiology reveals relations between network topology and physiological function". Nature Communications. 3: 702. arXiv:1203.0242. Bibcode:2012NatCo...3..702B. doi:10.1038/ncomms1705. PMC 3518900. PMID 22426223.
- Mani AR, Montagnese S, Jackson CD, Jenkins CW, Head IM, Stephens RC, Moore KP, Morgan MY (February 2009). "Decreased heart rate variability in patients with cirrhosis relates to the presence and degree of hepatic encephalopathy". American Journal of Physiology. Gastrointestinal and Liver Physiology. 296 (2): G330–8. doi:10.1152/ajpgi.90488.2008. PMC 2643913. PMID 19023029.
- Griffin MP, Moorman JR (January 2001). "Toward the early diagnosis of neonatal sepsis and sepsis-like illness using novel heart rate analysis". Pediatrics. 107 (1): 97–104. doi:10.1542/peds.107.1.97. PMID 11134441.
- Gholami M, Mazaheri P, Mohamadi A, Dehpour T, Safari F, Hajizadeh S, Moore KP, Mani AR (February 2012). "Endotoxemia is associated with partial uncoupling of cardiac pacemaker from cholinergic neural control in rats". Shock. 37 (2): 219–27. doi:10.1097/shk.0b013e318240b4be. PMID 22249221.
- Mølgaard H, Sørensen KE, Bjerregaard P (September 1991). "Attenuated 24-h heart rate variability in apparently healthy subjects, subsequently suffering sudden cardiac death". Clinical Autonomic Research. 1 (3): 233–7. doi:10.1007/BF01824992. PMID 1822256.
- Kloter E, Barrueto K, Klein SD, Scholkmann F, Wolf U (2018). "Heart Rate Variability as a Prognostic Factor for Cancer Survival - A Systematic Review". Frontiers in Physiology. 9: 623. doi:10.3389/fphys.2018.00623. PMC 5986915. PMID 29896113.
- Sutarto AP, Wahab MN, Zin NM (2012). "Resonant breathing biofeedback training for stress reduction among manufacturing operators". International Journal of Occupational Safety and Ergonomics. 18 (4): 549–61. doi:10.1080/10803548.2012.11076959. PMID 23294659.
- Goessl, V. C.; Curtiss, J. E.; Hofmann, S. G. (November 2017). "The effect of heart rate variability biofeedback training on stress and anxiety: a meta-analysis". Psychological Medicine. 47 (15): 2578–2586. doi:10.1017/S0033291717001003. hdl:2144/26911. ISSN 1469-8978. PMID 28478782.
- Miller EB, Goss CF (January 2014). "An Exploration of Physiological Responses to the Native American Flute" (PDF). arXiv:1401.6004. Bibcode:2014arXiv1401.6004M. Retrieved 25 Jan 2014. Cite journal requires
|journal=
(help) - Task Force of the European Society of Cardiology and the North American Society of Pacing and Electrophysiology (March 1996). "Heart rate variability: standards of measurement, physiological interpretation and clinical use". Circulation. 93 (5): 1043–65. doi:10.1161/01.cir.93.5.1043. PMID 8598068.