Cover's theorem
Cover's theorem is a statement in computational learning theory and is one of the primary theoretical motivations for the use of non-linear kernel methods in machine learning applications. The theorem states that given a set of training data that is not linearly separable, one can with high probability transform it into a training set that is linearly separable by projecting it into a higher-dimensional space via some non-linear transformation. The theorem is named after the information theorist Thomas M. Cover who stated it in 1965. In his own words,
A complex pattern-classification problem, cast in a high-dimensional space nonlinearly, is more likely to be linearly separable than in a low-dimensional space, provided that the space is not densely populated.
— Cover, T.M., Geometrical and Statistical properties of systems of linear inequalities with applications in pattern recognition, 1965
Proof
A deterministic mapping may be used: suppose there are samples. Lift them onto the vertices of the simplex in the dimensional real space. Since every partition of the samples into two sets is separable by a linear separator, the theorem follows.
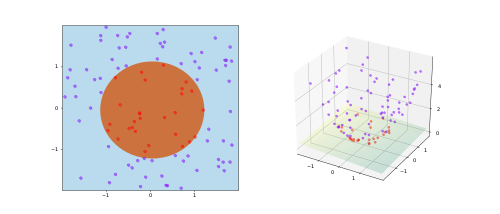
References
- Haykin, Simon (2009). Neural Networks and Learning Machines (Third ed.). Upper Saddle River, New Jersey: Pearson Education Inc. pp. 232–236. ISBN 978-0-13-147139-9.
- Cover, T.M. (1965). "Geometrical and Statistical properties of systems of linear inequalities with applications in pattern recognition" (PDF). IEEE Transactions on Electronic Computers. EC-14 (3): 326–334. doi:10.1109/pgec.1965.264137.
- Mehrotra, K.; Mohan, C. K.; Ranka, S. (1997). Elements of artificial neural networks (2nd ed.). MIT Press. ISBN 0-262-13328-8. (Section 3.5)