Average variance extracted
In statistics (classical test theory), average variance extracted (AVE) is a measure of the amount of variance that is captured by a construct in relation to the amount of variance due to measurement error.[1]
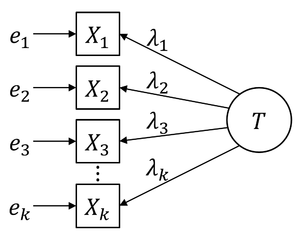
History
The average variance extracted was first proposed by Fornell & Larcker (1981).[1]
Calculation
The average variance extracted can be calculated as follows:
Here, is the number of items, the factor loading of item and the variance of the error of item .
Role for assessing discriminant validity
The average variance extracted has often been used to assess discriminant validity based on the following "rule of thumb": Based on the corrected correlations from the CFA model, the AVE of each of the latent constructs should be higher than the highest squared correlation with any other latent variable. If that is the case, discriminant validity is established on the construct level. This rule is known as Fornell–Larcker criterion. However, in simulation models this criterion did not prove reliable for variance-based structural equation models (e.g. PLS).,[2] but for covariance-based structural equation models (e.g. Amos) only.[3] An alternative to the Fornell–Larcker criterion that can be used for both types of structural equation models to assess discriminant validity is the heterotrait-monotrait ratio (HTMT).[2]
Related coefficients
Related coefficients are tau-equivalent reliability ( ; traditionally known as "Cronbach's ") and congeneric reliability ( ; also known as composite reliability) which can be used to evaluate the reliability of tau-equivalent and congeneric measurement models, respectively.
References
- Fornell & Larcker (1981), https://www.jstor.org/stable/3151312
- Henseler, J., Ringle, C. M., Sarstedt, M., 2014. A new criterion for assessing discriminant validity in variance-based structural equation modeling. Journal of the Academy of Marketing Science 43 (1), 115–135.
- Voorhees, C. M., Brady, M. K., Calantone, R., Ramirez, E., 2015. Discriminant validity testing in marketing: an analysis, causes for concern, and proposed remedies. Journal of the Academy of Marketing Science 1–16.